If you find an error of any kind on this page, or if you need an answer that is not here, or if the
explanation given here is insufficient, I am happy to help. Please send me an email request with
"Q&A Book" in the subject line using timcrack@alum.mit.edu.
The individual letter solutions to all Q&A Book Questions appear on pp. 269/270 of the Q&A book and also appear online at http://www.foundationsforscientificinvesting.com/TIIQ7-MC-ANSWERS.pdf (for any files at the web site that are password protected, please look up "password" in the index of FFSI or Q&A Book).
The answers below are numbered using the numbers in the 7th
edition of the Q&A Book. If, however, a question also appeared in the 6th edition, then that question number is given alongside the 7th edition number. For example, "Q2" gives the solution to Question 2 in the 7th and 6th editions, but "Q161/Q133" gives the answer to Q161 in the 7th edition (which is also Q133 in the 6th edition). If a Q&A book question appeared in the University of Otago FINC302 Mid-Term exam in 2020, then the MTQ number is given also. For example, Q140/2020MTQ5 gives the answer to Q140 from the 7th edition of the Q&A book (which also appeared as Q5 of the 2020 FINC302 Mid-Term exam).
Quoted page numbers are from the 10th Edition of
Foundations for Scientific Investing: Capital Markets Intuition and Critical Thinking Skills
(ISBN 978-0-9951173-6-5, December, 2020) (FFSI) unless otherwise indicated. Pages numbers in the 13th edition of FFSI (February 2024)
are roughly the same as for the 10th edition up to about p. 190, and then slowly deviate, with an additional 21 pages added by the
time you get to the end..
- Q2 Thank you for your question. I am happy to help. The KiwiSaver account earns 6% per annum. You must draw a time line here. There is no growing annuity here. It is a level ordinary annuity. My advice is to use PVA (present value of annuity) and add it to the lump sum at t=0, then compound everything forwards 65 periods.
I get PV=lumpsum+PVA=$2,000+(C/r)*[1-1/(1+r)^N]=$2000+(1,000/0.06)*[1-1/1.06^16]=$12,105.895.
Then FV=PV*(1.06)^65=$534,414, answer (c)
- Q3 This matrix dimension question runs us all the way back to Section 1.2.2 of FFSI, which was there to prepare you for the Markowitz efficient set mathematics in Section 2.6.4.
Anything with a little arrow symbol (called "\vec") is a column vector. It has dimension Nx1 let us say. N=20 here.
Anything with a little ' symbol had been transposed. So this swaps rows for columns.
As discussed on pp. 27-28, if you multiply two matrices, their inner dimensions must agree, and their outer dimensions tell you the size of the outcome. This is true for three matrices multiplied together.
So, A=(\vec i)'V^{-1}(\vec mu) is a (1xN) times an (NxN) times a (Nx1). The inner Ns agree, and the outer 1x1 tells us the size. Alternatively, you may simple recall that A was the 1x1 calculation I did for you to get you started on Q3.2.2 (Active Alpha Optimization; p. 254 Q&A Book).
(\vec H) and (\vec h_P) both have the little \vec symbol. So that are both Nx1 column vectors.
So, the answer is 1x1, Nx1, Nx1. With N=20 here.
- Q4 The answer to this appears in the Quant Quiz in the box on the bottom of p. 29 of FFSI. Remember that variance is of form h'Vh, so standard deviation must be the square root of that form.
- Q6 This numerical derivative question is similar to the Quant Quiz on p. 33 of FFSI, using the table on p. 35 of FFSI. The worked solution is on p. 34.
To calculate a numerical derivative, all you need are numerical values of the function. These values can come from a table of values, or these values can come from evaluation of the function.
In this case, note first that you can completely ignore the written function erf(x)=... because you have a printed table of numerical function values. So, you do not need to use the given formula for anything. If, however, there was a formula (presumably much simpler), but no printed table, then you would take the same approach as follows, but in that case you would use the formula (like 2020MTQ10).
So, in this case, I can just ignore the formula and look at the table. We need to find the slope, which is the first derivative. That is just slope=[f(x+h)-f(x)]/h for small h. We are asked for this at x=6.
Let me choose x=6 and h=0.01 (the smallest step from x=6).
I get slope=[f(x+h)-f(x)]/h=[f(6.01)-f(6)]/0.01=[0.9301596-0.9316814]/.01
=-0.0015218/0.01=-0.15218, answer (b).
This matters because we need to understand how Excel Solver is calculating slopes. We saw, for example, in
MIN-VAR-OBJ-REVISED.XLS that Solver calculates slopes using a very small step size, and it can be sitting at the minimum, but thinking it is at the maximum, because the slope is zero in all directions if you take only very small steps. This matters in
Q3.2.2 (Active Alpha Optimization; p. 254 Q&A Book)
- Q9 Student-t test of the mean. This is a review of our extended discussion of how to build a t-test of the mean in Section 1.3.16 and in the COCO spreadsheets*). It is also tied in with discussion of the general form of a t- or Z-test in Section 1.3.14. So, review those, ultimately leading you to specific Equation 1.55. Remember that every t-test has a slightly different form: t-test of mean is (sample mean-null hypothesis value (0 here))/(s/sqrt(N)), where s is sample stdev. We are using Equation 1.55 from p. 100 of
FFSI:
t=[Xbar - 0]/[sigma/sqrt{N}]
=[0.00064-0]/[0.013308/sqrt{500}]
=1.0753558, answer (b)
*COCO Spreadsheets:
- Q10 Student-t test of correlation (in this case auto-correlation). Tied in with discussion of general form of the test in Section 1.3.14, ultimately leading you to specific Equations 1.43 and 1.44 (latter equation only to be used in case where N is big and correlation is small). Like Q9, remember that every t-test has a slightly different form: t-test of correlation is (sample correlation-0)/[sqrt(1-correlation^2)*(1/sqrt(N-2))], or approximately (sample correlation-0)/(1/sqrt(N)), if correlation is small and N is big. One student asked me how to use the Yule factor here, but Q10 does not use a Yule factor. The Yule factor is used for adjusting the t-test of the mean, not the t-test of the autocorrelation.
See p. 107 of FFSI for discussion of this point.
Let us use the approximate formula first (Equation 1.44; it follows algebraically from the exact equation when the correlation is small and N is big):
t= (sample correlation-0)/(1/sqrt(N))=0.056773/(1/sqrt(500))=1.269 (answer (c))
Now let us use the exact formula (Equation 1.43)
t=(sample correlation-0)/[sqrt(1-correlation^2)*(1/sqrt(N-2))]=0.056773/[sqrt(1-0.056773^2)(1/sqrt(498))]
=1.269 (answer (c))
- Q12 Like Q6, note first that you can completely ignore the written function f(x)=... because you have a printed table of function values. To calculate a numerical derivative, all you need are numerical values of the function. These values can come from a table of values, or these values can come from evaluation of the function. So, you do not need to use the given formula for anything. If, however, there was a formula (presumably much simpler), but no printed table, then you would take the same approach as follows, but in that case you would use the formula (like 2020MTQ10).
A student asked me "In class the example function was x^3, and I can understand how this works. However in Q12 I am specifically confused with the function which is a much more complicated, function: f(x) = sin(x · pi)-Gamma(x,pi) where Gamma(x,pi)… I’m aware that the process is the same, so I only have difficulty with applying the steps to the more complicated functions of x." The student was mixing up two concepts. This is a valuable mistake because we can all learn from it. Q12 asks for an estimate of the derivative to this function using numerical techniques. You are not looking for the exact analytical derivative.
We did not estimate the derivative for x^3 in FFSI; we found an exact analytical derivative in that case. These are two different concepts. Look at the quant quiz "first derivative"
bottom half of p. 33 of FFSI for an estimated derivative using numerical techniques. To find slope, you evaluate the function at two values very close together, and divide by the step between them: [f(x+h)-f(x)]/h. In this case, we are finding the slope at x=7, so I used I used
slope=[f(x+h)-f(x)]/h
= [f(7.01)-f(7.0)]/0.01.
= [-0.996766- (-0.965099)]/0.01
=-0.031667/0.01=-3.1667, answer (a)
Ask again if not clear.
- Q13 You can remove the words "small-cap" and "large-cap" from this question, if you wish, and the answer does not change. The only reason I included these was for emphasis. On pp. 105-106 of FFSI,
I told you that small-cap stocks require large samples (e.g., N=200+) and large-cap stocks require smaller samples (e.g., N=100+) in order for the central limit theorem to overpower the non-normality of underlying data and allow the t-statistic for the mean to be valid. By stating the market cap, I was emphasizing that 30 is definitely too small and 500 is very likely big enough.
- Q14 Oh, that's interesting. When you see prices bouncing like this, they are bouncing between the bid and the ask (see pp. 214-215 of
FFSI). This is a US stock, supported by a market maker, but we see similar patterns in NZ stocks. B, D, and F look like a customer buying at the ask price (think of it as being like a naive market order to buy hitting the ask in the NZX CLOB). Trades A and G look like a customer selling at the bid price (think of it as being like a naive market order to sell hitting the bid in the NZX CLOB). I cannot tell what trade E is; I would have to go back the Bloomberg terminal and change the little box that says "Trade" to "Bid" or to "ask" to see whether that was the bid or ask. Trade C must be a case of US price improvement. The only answer I like is Answer (b).
- Q15 Using the same logic as Q14, none of these answers makes sense. It must be (e).
- Q16 Yes, the bid-ask spread is $0.01 wide when trades A, B, C, and D occur. That's just the distance between the bid and the ask. I had to read the $0.01 off the vertical scale. Yes, the bid-ask spread is $0.01 wide when trades F and G occur. Yes, Trade C likely involves price improvement. Yes, we cannot immediately tell whether trade E was a customer buy or customer sell, because it is not obvious whether trade E takes place at the ask price just after the market maker dropped the level of the spread from 2.06-2.07 to 2.05-2.06, or whether trade E takes place at the bid just before the market maker dropped the level of the spread from 2.06-2.07 to 2.05-2.06. So, answers (a), (b), (c), and (d) are all true. I was looking for a false answer, so I am left with answer (e).
- Q17 I told you in Chapter 1 (Figure 1.18 p. 88) it would be non-linear, and we analyzed the relationship using two correlations: PPMCC (which assumes a linear relationship) and SROCC (which assumes generally increasing or generally decreasing relationship, but not necessarily linear). Answer (a) says OLS, but that assumes linearity, and the R^2 tells us how close to linear it is. Answer (b) says look at the PPMCC, but that assumes linearity (and PPMCC^2=R^2 for that reason). Answer (c) is just a rescaled version of Answer (a). If it wasn't linear in (a) it's not linear in (c). So, I think I would plot the data, and maybe use SROCC (if it were generally increasing or generally decreasing). In practice, in larger samples, stdev of returns tends to fall with rising market cap, but our 21-stock sample is too small to reveal it confidently.
- Q18 We are using Equation 1.55 from p. 100 of FFSI:
t=[Xbar - 0]/[sigma/sqrt{N}]=[-0.000327-0]/[0.017007/sqrt{500}]=-0.429937, answer (c).
- Q19 Student-t test of correlation (in this case auto-correlation). Tied in with discussion of general form of test in Section 1.3.14, ultimately leading you to specific Equations 1.43 and 1.44 (latter equation only to be used in case where N is big and correlation is small).
Let us use the approximate formula first (Equation 1.44): t= (sample correlation-0)/(1/sqrt(N))=-0.092763/(1/sqrt(500))=-2.074 (very close to answer (b))
Now let us use the exact formula (Equation 1.43):
t=(sample correlation-0)/[sqrt(1-correlation^2)*(1/sqrt(N-2))]=-0.092763/[sqrt(1-(-0.092763^2))(1/sqrt(498))]=-2.079 (answer (b)).
- Q20 This takes us back to conformal matrix multiplication examples on p. 27/28 of FFSI. A matrix has dimensions 2x3, for example, if it has 2 rows and three columns, like matrix B in Equation 1.4 on p. 28 of FFSI. If you multiply a (kxn) matrix by an (nx1) matrix, then the two matrices are conformal. That means that their inner dimensions (n here) are the same. If you multiply two matrices together, you know the size of the answer by looking at the outer dimensions. So, for example, if you multiply a (kxn) matrix by an (nx1) matrix, the answer is of dimension (kx1). There are two ways to answer this question. First, an informed answer with no real algebra involved. Second, a tedious step-by-step approach where we work out the dimension of each item in each multiplication, and then combine dimensions using the rules for conformal matrix multiplication just mentioned.
- First approach (my favorite): The first term [(mess)V^{-1}(mu - Rfi)] is named in the question as hp with a little vec symbol on it. So, we know that symbol means it is an Nx1 column vector. N=20 here, so this must be 20x1. The second term [V^{-1}(mu - Rfi)] is just pulled from the first term. The item I called (mess) must be a 1x1 scalar because mu_P and RF in the numerator of that mess are 1x1. You can always multiply a vector or matrix by a scalar, so in the expression [(mess)V^{-1}(mu - Rfi)], the first multiplication (mess)V^{-1} is scalar multiplication (which would just use "*" in Excel) but the second multiplication V^{-1}(mu - Rfi) is matrix multiplication (which would use MMULT in Excel). So, given that this "(mess)" term is just a scalar, the [V^{-1}(mu - Rfi)] term must have the same dimensions as the full [(mess)V^{-1}(mu - Rfi)] term, that is, 20x1. Giving answer (d).
- Second approach (more tedious, and not really needed): the first item in square brackets looks like [(mess)V^{-1}(mu - Rfi)] We know that V is always the (NxN) square variance-covariance matrix. We know that mu here is an (Nx1) column vector because that's what the little vec symbol on top means, and the same for the i term. The item I called (mess) must be 1x1 because mu_P and RF in the numerator of that mess are 1x1. So, we have (1x1)(NxN)(Nx1). That looks like it is non-conformal because the inner dimensions (1x1)(NxN) do not agree, but, that's scalar multiplication there. Like this: =(1x1)*MMULT(NxN,Nx1). That is, the first (mess) term is just a 1x1 number that multiplies everything that follows. So, we need only look at the dimensions of the MMULT(NxN,Nx1) term, which we get from the outer dimensions: Nx1. The second term [V^{-1}(mu-RFi)] is just like what we just worked out, for the same reasons: Nx1. N=20 here, so, I am looking for 20x1 and 20x1, answer (d).
- Q22 You have Equations (1.43) and (1.44) (the first two correlation t-statistics formulae on p. 95 of the book). The denominator is the standard error estimate. The first equation (1.43) yields sqrt{1-rho^2}/sqrt(N-2)=sqrt(1--0.04^2)/sqrt(498)=0.044775, and the second equation (1.44), which is an approximation, yields 1\sqrt{N}=0.04472. I did not know which you would use, so I asked for an answer "close to" my given number. Keep in mind, from Equation 1.40 in Section 1.3.14 ("Intuition for General Functional Form of Z and t Tests") that Z and t tests always have the form [parameter-null value]/[standard error]. So, you can always pick the standard error out of the denominator.
- Q23 (see also Q46) You can almost always reject normality of returns for stocks (because of fat tails and peakedness in the distribution, relative to the shape of a normal distribution with the same mean and variance). So, we are looking for a number at the high end of the scale. 90% is the BEST answer here, though in the Otago University 2020 dataset, my students rejected normality in 100% of their stocks.
- Q24 Mean blur implies that you often cannot reject the null hypothesis that the mean return is zero. You can, however, reject it sometimes. So, I am looking for an answer that is small, but not zero. Answer (d) says a "small proportion". So, that is the best answer. In exercises in 2020, my students could reject the mean return of zero in six out of 21 stocks. This was an unusually high proportion and is attributable to a roaring bull market, with low volatility (during your sample period).This is not the normal state of affairs. In normal times, you would reject the mean of zero in maybe 2-3 stocks out of 21.
- Follow up student Question: You said that we "often cannot reject the null hypothesis that the mean return is zero." I do not fully comprehend this, so does it mean that the mean return is zero?
- Answer: No, it does not mean that the mean return is zero. Mean blur is when the mean return is a small number relative to the standard deviation of returns. In exercises that my students did in 2020, they saw that the average ratio of standard deviation of returns to mean returns in their 21 stocks was about 50. That is, the variability of returns is very high compared with the mean return. That is, a t-statistic for the mean will often be a small number (because it has mean in the numerator and standard deviation in the denominator). Sometimes the mean return on a stock is positive, sometimes the mean return is near zero, sometimes the mean return is negative. The problem with the high standard deviation of returns on stocks is that it is difficult to distinguish between these cases, because there is so much variability in the data. This means that even if the mean return is positive, we often cannot reject the null hypothesis H0:mu=0.
- Q25 We discussed this on p. 80 of FFSI. We declared that the correlation would be in the region of 95% to 99%, more or less. Answer (b) is the only answer that comes close. Answer (a) is not sensible; these correlations cannot be bigger than 1. If the correlation were exactly 1 (a student asked), that would mean that a plot of P(t-1) versus P(t) is a perfect straight line. There is an interesting mathematical reason why you cannot have that and the correlation would not be defined. Ask me some other time.
- Q27 The t-test of the mean has three assumptions: normality, independence, and identical distributions for the underlying data. Yes, answer (b) is correct. One month is about 21 observations. A sample of 21 is not enough to enable the CLT to kick in and override the effect of the significant non-normality (see pp. 105-106 of FFSI). The small adjustment using the autocorrelation coefficient is not about correcting for non-normality. It is about correcting for auto-correlation, which is one form of dependence. It is discussed on p. 107 of FFSI. It does not apply here.
- Q28 Student-t test of the mean. This is a review of our extended discussion of how to build a t-test of the mean in Section 1.3.16 and in the COCO spreadsheets*. It is also tied in with discussion of the general form of a t- or Z-test in Section 1.3.14. So, review those, ultimately leading you to specific Equation 1.55. Remember that every t-test has a slightly different form: t-test of mean is (sample mean-null hypothesis value (0 here))/(s/sqrt(N)), where s is sample stdev. My students had a question on this in their 2020 Mid-Term exam (2020MTQ20). We are using Equation 1.55 from p. 100 of FFSI:
t=[Xbar - 0]/[sigma/sqrt{N}]
=[0.001436-0]/[0.0181796/sqrt{500}]
=1.76626, answer (c)
*COCO Spreadsheets
- Q29 Student-t test of correlation (in this case auto-correlation). Tied in with discussion of general form of test in Section 1.3.14, ultimately leading you to specific Equations 1.43 and 1.44 (latter equation only to be used in the case where N is big and correlation is small).
Let us use the approximate formula first (Equation 1.44):
t= (sample correlation-0)/(1/sqrt(N))=0.0252561/(1/sqrt(500))=0.5647 (answer (d))
Now let us use the exact formula (Equation 1.43)
t=(sample correlation-0)/[sqrt(1-correlation^2)*(1/sqrt(N-2))]=0.0252561/[sqrt(1-0.0252561^2)(1/sqrt(498))]=0.5638 (answer (d))
- Q30 Interesting. I showed this picture of NZX relative spreads during the trading day in my classes in 2019 and 2020. The PPMCC is for linear relationships. The relationship has been described as U-shaped. So, that is not linear. So, the PPMCC is not going to capture the full relationship. The SROCC is for monotonic relationships. That is, relationships of a mono (i.e., single) tone (i.e., manner). The SROCC is for generally increasing or generally decreasing relationships. The relationship has been described as U-shaped. So, that is not a single manner. It is neither generally increasing, nor generally decreasing. So, the SROCC is not appropriate either. What you really need to do is fit something like a parabola to it, and then use non-linear least squares to see how good the fit is. This is not something we have talked about.
- Q31 See also Q40 and Q125. The ratio of variances is an F-test, as long as the samples (and thus their variances) are independent, and the underlying data are independent and identically normally distributed. We looked at the F-test on p. 59 (constructive demonstration applying very generally), on p. 63 (relationships between distributions summarizing the previous), p. 103 (specific construction corresponding to this question, following on from part of the t-test construction).
- Q35 This one is very similar to the Quant Quiz on p. 96 (with worked solution there). We have a t-statistic formula Equation 1.43 but I do find it messy to work with. So, given that the correlation is relatively small, I think the sample size will have to be big for it to be significant. So, let us instead use the approximation formula Equation 1.44. That one says t=rho/[1/sqrt(N)], where "rho" is the correlation. That simplifies to t=sqrt(N)*rho. That will be much easier to work with.
Let me point out a sneaky way to do this without any algebra. Just use t=sqrt(N)*rho directly, and try N=500 (answer a), then N=1400 (answer b), until you find the answer! Answer (a) was too small, but (b) did the job. Then you are done.
...but by long hand, when N is large, the t distribution looks like a standard normal distribution. So, a test significant at the 5% level means the t-stat will be 1.96 (plus or minus).
So, I am going to solve 1.96=|t|, for N, where |t| is absolute value.
Algebra: 1.96=|t|=sqrt(N)*|rho| implies that sqrt(N)=1.96/|rho|=1.96/0.0532845=36.78. Square both sides to get N=1,353. Rounding up to the nearest 100, I get 1,400, answer (b).
- Q37 Delta = the derivative of option price with respect to stock price. Or, in other words, Delta = the slope of the option pricing function. So, you need to estimate slope. Q6 and Q12 are similar, though without the option pricing context. You evaluate the function at two values very close together, and divide by the step between them: [f(x+h)-f(x)]/h. In this case, I used [f(5.01)-f(5.0)]/0.1. In this exercise you have a table to give you option prices. This is discussed on p. 33 and p. 34 of FFSI. Please review FFSI and try again. Just like Q6 and Q12. This time I did not give the function, but I can tell you it looks like the Black-Scholes formula for a European-style put option to me. I need to evaluate the function at two values very close together, and divide by the step between them: [f(x+h)-f(x)]/h. In this case, we are finding the slope at x=5, so I used I used
slope=[f(x+h)-f(x)]/h
= [f(5.01)-f(5.0)]/0.01.
=[0.2720-0.2766]/0.01
=-0.0046/0.01=-0.46, answer (b).
- Q39 This is a guess really, based on our observation that having perfect foresight 1% of the time added 1% per annum to our rate of return. So, 50.5%-49.5% is a 1% of the time advantage.
- Q40 F-tests appear on p. 103 of FFSI, building upon the earlier work in the t-stat argument (and also the ping-pong ball argument on p. 59). So, all you need is a ratio of sample variances: F=0.025^2/0.015^2=2.78
- Q42 Read the answer to Q20 before trying this. As argued in Q20, hP with the vec symbol is an Nx1 column vector (that's what the vec symbol means here). So, we immediately know that hP= [(mess)V^{-1}(mu - Rfi)] must be Nx1 (looking at the left-hand side and completely ignoring the right-hand side), and N=20 here, so [(mess)V^{-1}(mu - Rfi)] is 20x1. As argued in Q20 [(mu - Rfi)V^{-1}(mu - Rfi)] is [(Nx1)'(NxN)(Nx1)]=[(1xN)(NxN)(Nx1)], remembering that the transpose of that first term swaps rows and columns. Then the dimension of this can just be read off the outer dimensions: (1x1). So I get answer (a).
- Q43 Contrast this question with Q4. The Tobin frontier is obtained by investing in the risky assets plus investing in the riskless asset.
For the Tobin frontier, the vector hP(i.e., the weight of the investments in the risky assets), need not add to 100%. For example, if you put half your money in the risky assets and half in the riskfree asset, then hP adds to 50% and hP alone does not determine your return. Because half you money is in the riskless asset in this case, you need to bring RF into it to account for the return from the part of your money in the riskfree asset.
So, for the Tobin frontier we have mean = hP' mu + (1-hP'i) RF. How do you read that? Well hP' mu is the return from the risky assets, as usual, and hP'i is the vector of portfolio weights times that vector of ones we used Q3.2.1 (Markowitz; p. 251 Q&A Book) and Q3.2.2 (Active Alpha optimization; p. 254 Q&A Book); All that hP'i does is add up the weights. So, for example, suppose there are four stocks, and suppose hP=(0.25 0.25 0.00 0.00)'. Then you have 25% in stock 1, 25% in stock 2, nothing in stock 3, and nothing in stock 4 (the other half of your money is assumed to be invested in the riskless asset). Then mean =hP' mu + (1-hP'i) RF is given by [(0.25*mu1)+(0.25*mu2)+(0.00*mu3)+(0.00*mu4)] +[(0.25*1)+(0.25*1)+(0.00*1)+(0.00*1) ]RF which yields (0.25*mu1)+(0.25*mu2) +0.50*RF. That is, your mean return is 25% times the mean return on stock 1, plus 25% times the mean return on stock 2, plus 50% (the balance of your investment) times the return on the riskless asset. That seems to make sense if 2% of our money is in stock 1, and 25% of your money is in stock 2, and 50% of your money is in the riskless asset.
Note that in the special case where the Tobin Frontier portfolio P=T (i.e., the Tangency Portfolio),
the portfolio is fully invested. In this case only, the RF component does not appear. In this case only,
Answer (a) is correct. Otherwise, Answer (a) is false.
TEST: What if RF=0? Is Answer (a) correct all the time then? I hope you answered "no."
- Q45 (see also Q83) I built this question purposely because many students (you are not alone by any means!) were confusing two different concepts. Let me explain and add another comment.
First, if there is significant excess kurtosis (and there often is!) then your data are not normally distributed. End of story. Sample size is irrelevant. Either the data are normally distributed or not normally distributed, and significant excess kurtosis (or significant skewness) is enough to reject normality. So, answer (b) is correct.
Second, the Student-t test of the mean is built on three assumptions: your data are normally distributed, your data are statistically independent of each other, and your data are identically distributed. I can prove, however, using some probability theorems and two pages of detailed algebra, that if you have a large enough sample, say over 200, then the first assumption is no longer needed, as long as the other two assumptions hold. That is, even if your data are not normally distributed, you can still compare your calculated Student-t statistic for the mean to the Student-t tables.
Note that in neither of the above cases does anything happen that makes the data normally distributed. So, answer (d) is not correct, although the second half of the sentence in answer (d) is correct in the case of a Student-t test of the mean, which is not mentioned anywhere in the question.
Let me add one other comment. In the case where the sample size is large, the Student-t random variable behaves like a standard normal random variable. So, when conducting a t-test in a large sample, you can just compare your test statistic to Z (i.e., standard normal) tables.
- Q46 (see also Q23) You can almost always reject normality of returns for stocks (because of fat tails and peakedness in the distribution, relative to the shape of a normal distribution with the same mean and variance). So, we are looking for a number at the high end of the scale. Answer (a) says "most if not all, say nine or 10" and is the BEST answer here, though in the Otago University FINC302 2020 dataset, my students rejected normality in 100% of their stocks. Q53 was about mean blur, but Q46 is about non-normality, and especially kurtosis. My students tested for normality in 2020 using 21 stocks and rejected normality for every one of the 21 stocks. The rejections were very strong, and driven mostly by excess kurtosis (i.e., peakedness and fat tails relative to a normal distribution with the same mean and variance). This is a standard result in finance. We very often reject normality of returns. So, the answer must be (a), we will reject normality for most, if not all, of them. Figure 1.16 on p. 71 of FFSI is a nice illustration of this; keep in mind, however, that I truncated this figure. That is, although you can see the peak near zero (caused by lots of small returns during calm periods) the figure actually extends to about 11% or 12% on the right-hand side and to -20% on the left-hand side (i.e., fat tails with extreme events).
- Q47 I use the approximation formula Equation 1.44. I get t=rho/(1/sqrt(N))=0.0668/(1/sqrt(504))=1.4996. That leads me to answer (b).
- Q51 This ties in with discussion of correlation and prediction on p. 80 of FFSI "Correlation Example with Actual Stock Prices". The short answer is that you can predict prices really well using lagged prices (so, b = 1, roughly), but that's not where the money is. You cannot predict returns easily at all (so, d = 0, roughly), and that is where the money is.
- Q53 is about mean blur. Out of every 10 stocks there are usually only "a few" for which the mean return is significant, given the high variability. Note also that this is about the first and second moments (mean and variance) and has little or nothing to do with the third or fourth moments (skewness and kurtosis).
- Q54 is about p. 80 of FFSI. P(t-1) is a very good predictor of P(t). You can test this yourself using prices (without any missing observations). P(t-1) is such a good predictor of P(t) that the R^2 is going to be very close to 1. Ask yourself, if I tell you that Microsoft (MSFT) closed at $187.74 per share this morning, what is a very good guess of where it will close during the next trading session? Well it could go up a little, and it could go down a little, so, on average, over time, if you guess that its next closing price is the same as its most recent closing price, on average you will be very close to being correct. A price process where your best guess of tomorrow's price is today's price is called a "martingale," which you may come across in FINC306 (i.e., our derivatives class).
- Q56 (see also the Quant Quiz on p. 108 of FFSI) We know it is chi-squared because it is independent squared standard normal terms added up. There are 45 such independent terms, so there are 45 degrees of freedom. This follows directly from the definition of a chi-squared random variable given on p. 58 of FFSI.
- Q62 Look at Figure 1.7 on p. 42. You can see that that little hatched rectangle of width wi is only an approximation to a lump of probability mass (i.e., you can see those corners at the top are not meeting the density function). In fact, you can see that wi is, maybe, 5mm wide in the figure. That is, wi is non-infinitesimal. When the integral is written with the "dx" in it, then yes, that "dx" is an infinitesimal quantity. When the integral is written as an approximate summation involving, in this case, heights hi and widths wi of little boxes, and values of xi, then that little width wi is not infinitesimal. That's why that summation is an approximation to the value of the integral. The key is that as we let wi go to zero (so the rectangles get narrower and the count of them increases), then in the limit as wi goes to zero, our approximation becomes more and more accurate. So dx is the limiting value of wi, when wi has gone to zero.
- Q63 (see also Q113 andQ134/2020MTQ22) Load up the spreadsheet
Q63-Q113-20200610-REVISED.xlsx
and hit F9 a few times to watch the simulation. I used Equation 2.2 (geometric Brownian motion RW) to generate the continuously compounded returns (CCR) r(n)=mu*tau+sigma*sqrt(tau)*z(n) (with tau=time step) and then I used P(n+1)=P(n)*exp(r(n)) to generate prices. With correlation questions like this, always go back to basics. The correlation is the covariance divided by a product of standard deviations, like in Equation 1.41 on p. 93 of FFSI. So, the correlation takes its sign from the sign of the covariance in the numerator. So, it comes down to the sign of the covariance. Now go back to basics again. The covariance is given by Equation 1.36 on p. 84 of FFSI. It is a product of moments. So, the sign of covariance is determined by whether the two terms (X-mean) and (Y-mean) have the same sign or different sign. If both X and Y tend to be above their means at the same time, and below their means at the same time, then you get positive*positive and negative*negative, both of which yield a positive covariance and thus a positive correlation. If, however, X tends to be above its mean when Y is below its mean, and vice versa, then you get positive*negative and negative*positive, both of which yield a negative covariance and thus a negative correlation. The description of prices and returns in this question is enough to deduce the signs here, and you can see it in action in the spreadsheet simulation.
- Q64 Kurtosis is both peakedness and fat tails relative to a normal distribution. Figure 1.16 shows that there are many more small returns (i.e., peakedness) and many more tail events (i.e., fat tails) than in a normal distribution with the same mean and variance. Table 1.7 shows that there are many more tail events than in a normal distribution with the same mean and variance (e.g., 2020:Q1). Table 1.8 shows that there are many more small returns than in a normal distribution with the same mean and variance (e.g., as experienced during all of 2017). Discussion in the nearby text on pp. 68-73 of FFSI gives further details.
- Q67 The t-test of the mean tests a hypothesis about the value of mu, the population mean. The null hypothesis is often H0:mu=0. This is not a test of whether the data are normally distributed or not. It is only a test of whether mu=0 or not. If I reject H0:mu=0, then maybe the data are normally distributed, but with a higher mean than I thought. If I want to test normality, I use a Z{skew} or Z{kurt} or JB=Z{skew}^2+Z{kurt}^2 test, but there is no information about testing skewness or kurtosis here, so there is no information about normality. So, the answer is (d).
- Q73: I had forgotten about Corrections Corp. Their stock has done about 40% worse than the S&P500 over the last year (to May 6, 2020), but with a lot of stock-specific risk. The key here is to understand correlation and R^2. First of all, R^2 = correlation^2. So, correlation = -0.80 implies R^2=0.64. That knocks out two possible answers. Next, the sign of the correlation is the same as the sign of the slope. So, negative correlation means downward sloping. That knocks out one more answer. Lastly, correlation of -0.80 is quite large, and so is R^2=0.64. So, the line of best fit is going to be clear. Only if correlation = 0 would we get a big round ball of points. So, that knocks out one more answer, leaving (d).
- Q82 This picture is Figure 1.16 on p. 71 of FFSI, and is discussed on pp. 68-73. It says there that there are many more observations in the tails than we would expect in a normal distribution. We saw many such observations during the 2020:Q1 covid panic. Thus (a) is the only correct answer.
- Q83 (see alsoQ45) The question is about whether the t-statistic for the mean is valid or not. The Student t-statistic for the mean has three assumptions: the data are normally distributed, the data are statistically independent of each other, and the data are identically distributed (so their parameters are stable). If any one of these assumptions does not hold, then you have to ask yourself if the test is valid. We get lucky in the case of non-normally distributed data. As long as you have a large enough sample, and the other two assumptions are not violated, then I can prove (using a central limit theorem, and Tchebychev's inequality and Slutsky's theorem) that the Student t-statistic for the mean is still a valid test. This is discussed on pp. 104-106 of FFSI. So, how large is large enough? It turns out that about N=100 is enough in large-cap stocks and N=200 is large enough in most small-cap stocks. We have N=750 in this question, and no other violations of assumptions. So, the test is valid. Answer (c)
- Q86 A student asked why is N=20 and not N=40. It is because each observation here is a pair. Imagine plotting these points on an X-Y graph to see how close they are to a straight line (which we did often). Each point requires a pair (R(IBM), R(MSFT)). So, you end up with only 20 points. This N=20.
- Q87 Q3.2.2 (Active Alpha Optimization) appears on p. 254 of the Q&A book. See also Q238 which includes a plot of similar data. See also top panel of Figure 1.18 on p. 88 of FFSI (relationship not quite as strong because 500 stocks obscures it a little; stronger results hold in NZ data with fewer stocks).
- Q93 Average dividend yield over the 50-year S&P500 sample was roughly 3.1% (This is exactly the same number as the average dividend yield in a 21-stock sample from the NZX I used in class in 2020; although average NZ dividend yield is usually higher than average US dividend yield, these samples are from different time periods, and average US dividend yields have fallen by 2020 to roughly 2%, and by 2021 to roughly 1.6%). The 3% number is discussed on p. 9 of FFSI. It is a standard number that you should have in your head, but you should be aware of where we are now also.
- Q96 The Spearman rank-order correlation coefficient (SROCC) is strongly negative. The SROCC detects relationships that are generally monotonic. That is, generally upward sloping, or generally downward sloping. In this case, we know that the relationship is generally downward sloping and strongly so. That is all we know. It could be linear, it could be non-linear. We do not have any additional information to help tell us whether the relationship is linear or non-linear. Also, the relationship is strong, not weak. So, each of answers (a), (b), (c), and (d) is false. If this question had said that the traditional Pearson product moment correlation coefficient (PPMCC) was equal to -0.91, then we would know that the relationship was strongly negative and linear, but that is not what we are told here.
- Q98 (see also Q107) This is about OLS and about R^2 just being the correlation squared. Let me review this concept using another important item as an exemplar:
PLOT A* is given here:
PE-GREPS-OEX-2019.pdf. It shows P/E ratio on one axis and analyst estimates of future growth rate in earnings per share on the other (let me call that growth rate "g(EPS)"). The data are the sub-sample of the 100 largest stocks from the US S&P500 index (called the S&P100, or the "OEX"). I argue that the higher the forecast g(EPS), the higher the P/E, and that in theory the slope of the relationship should be about 1, which is what I find. Look at the OLS R^2 of 44%. Can you tell me the correlation between P/E and g(EPS)?
Well, in the old days, correlation was always denoted "r" or "R". I think because it was originally called co-relation, and the r stood out more than it does now. Nowadays, instead of the Latin letter r we usually represent correlation using the Greek equivalent rho, which looks a bit like a little p with its tail off to the right a little. It is the same letter, pronounced as an r, but written with a different symbol in Greek.
To cut a long story short, R^2 is just r squared. In other words, if the R^2 is 44%, get your calculator out and square root it to find that the r (or rho, or correlation) is sqrt(.44) which is 66%.
...but there is a catch. You know how sqrt(4) can be +2 or -2? Well the square root of the R^2 also has a sign. The sign is the sign of the slope of the OLS regression. In my P/E vs g(EPS) case, the slope is positive. So, the correlation is +66%. ...but if that line had been downward sloping, the correlation would have been -66%. Note, of course, that both +66% and -66%, when squared, give an R^2 of 44%.
Incidentally, 44% is unusually large for an R^2 in finance. So, that's a good fit.
Digest this, then go and try Q98 and Q107 again, with your calculator in your hand and awareness of slopes. If you know R^2 = 95%, and you know the slope is positive, then the correlation is positive, but if you do not know the sign of the slope, then you are stuck. You don't know whether the correlation is positive or negative.
*PLOT A This OLS line of best fit shows that P/E and forecast g(EPS) are related. So, thinking about P/E as a reflection of forecast growth rates in EPS is justified. R^2=44% implies correlation 66%. [Details: Plot drawn May 5, 2019 showing Bloomberg's BEst_PE_RATIO versus BEST_EST_LONG_TERM_GROWTH for the OEX (S&P100) stocks, where BEST_EST_LONG_TERM_GROWTH is described thus "Long Term Growth Forecasts are received directly from contributing analysts, they are not calculated by BEst. While different analysts apply different methodologies, the Long Term Growth Forecast generally represents an expected annual increase in operating earnings per share over the company's next full business cycle. In general, these forecasts refer to a period of between three to five years."]
- Q99 On Monday I spend $10,000: $6,000 to buy 5 shares of PCLN and $4,000 to buy 1,000 shares of CHK. Those counts of shares are very important here.
On Tuesday, PCLN goes up $30 a share (to $1,230) and CHK goes down $0.25 per share (to $3.75). My portfolio is now worth 5*1230 + 1000*3.75=6150+3750=$9,900.
On Wednesday, PCLN goes up $30 a share (to $1,260) and CHK goes down $0.25 per share (to $3.50). My portfolio is now worth 5*1260 + 1000*3.50=6300+3500=$9,800.
So, from Tuesday to Wednesday, the value of my portfolio goes from $9,900 to $9,800. The return is (final-initial)/initial = (9800-9900)/9900=-0.010101, answer (a).
For me, the key was counting the number of shares. There are other ways to do it. You might have noticed that you gained $150 on PCLN and lost $250 on CHK, giving a loss of $100 on the first day. Similarly, there was a loss of $100 on the second day. So, they you could say your $10000 went to 9900 and then 9800, and then find the return.
- Q100 I particularly like this one, because I published a paper on it with a hedge fund manager. Remember that we discussed t-statistics for the mean in that detailed Section 1.3.16, based on several assumptions. The next section, 1.3.17 asked what if the assumptions don't hold. That's important, because in finance the assumptions often do not hold! There are three assumptions: normality of returns (we know that's not true!), independence of returns, and identical distributions (i.e., stable mean and variance through time). I discussed violations of these assumptions one at a time on pp. 104-108. On p. 107 I say that based on Crack and Ledoit (2010), if the only violation is that you have autocorrelation rho, then all you have to do to correct for this violation of the assumptions of the t-test is to multiply your regular t-statistic by (1-rho). So, in this case, we would get the corrected t-stat = t*(1-rho) = 2.4 * (1-0.25)=1.8, answer (b). In this case, the t-stat goes from being significant to insignificant, and so it really matters.
Here also is my answer to the very similar 2020MTQ23. There is a formula for this (and you can jump down to the bottom of this fat paragraph to see it, but the details are important). Just after we discussed the construction of the t-test of the mean (Section 1.3.16), we discussed (on pp. 104-108 of FFSI) robustness to the three assumptions: normality, independence, identically distributed. On pp. 106-107 we discuss what happens if the independence assumption is violated. The presence of auto-correlation is a violation of the independence assumption because it says that successive returns are statistically related to each other. Near the bottom of p. 106 there is a formula with a "*" beside it. It involves a covariance term, and on the next line the covariance is written as a correlation*stdev1*stdev2. The usual formula for the t-statistic assumes that this correlation between successive returns is zero. So, these cross-product correlation terms do not usually appear. If, however, there is auto-correlation of -25% (as there is in this question) then once you take these (in this case negative) terms into account, the revised/corrected standard error for the t-statistic gets smaller (i.e., it is decreased by these negative terms). The standard error sits in the denominator of the t-statistic (see Equation 1.40 on p. 92). So, if this gets smaller, then the t-statistic as whole gets bigger. It is a very complex calculation, but I published a paper in 2010 with hedge fund manager, mentioned in the middle of p. 107, that argues that a very good approximation to the complex calculation is to simply multiply the original t-statistic by (1-rho), where rho is the auto-correlation. In this case, the revised t-statistic, that accounts for the auto-correlation is given by t*(1-rho)=1.80*(1-(-0.25))=1.80*1.25=2.25, answer (d). If the auto-correlation were positive, then the revised t-statistic would instead get smaller by factor (1-rho), because of a larger standard error.
- Q103 Look at the bottom of p. 5 of FFSI. You will see that over the 50-year period, $1 grew to $108.45 in stocks, but only $13.25 in T-bills. The ratio is mentioned there as being close to 8.
- Q107 (see also Q98).
- Q111 In Chapter 1 we emphasized that you only needed to have perfect foresight 1% of the time to add 1% to the annual return over that 50-year period (which then adds half as much again to your ending wealth). This means you need only be perfectly insightful 1 trading day out of every 100 trading days. There are about 21 trading days a month, so that is one day out of every five months. This is discussed on p. 8 of FFSI. [One of the reasons this matters is that if it really takes so little skill to add 1% per annum, we need to ask why so called "skilled" managers underperform their benchmarks so much of the time. We will see they do terribly over the long run when we discuss the SPIVA results in Section 2.14.8.]
- Q112 This has two diamonds on it because most students do not take the time to look at the basic relationship between correlation, beta, and R^2. Also, we tend not to teach this in stats classes, even though it is not difficult. This is similar to Q33 on the 2020 Otago University FINC302 mid-term exam (Q148/MTQ33). The hint given in Q148/MTQ33 is to look at the formula for beta and the formula for rho (correlation). If you compare the two formulae, you will see that they are every close. The simple relationships are corr(X,Y)=cov(X,Y)/[std(X)std(Y)], beta(X,Y)=cov(X,Y)/[std(X)std(X)] (beta of Y relative to reference X), and R^2(X,Y)=corr(X,Y)^2. So, if std(X)=std(Y), then beta(X,Y)=corr(X,Y) because the denominators are the same. In our case, we are regression X=R(t) on Y=R(t-1), so std(X)=std(Y) almost perfectly, especially in a large sample, so we immediately get that (a), (b), and (c) are true. Answer (d) is true by definition in this case because they are the same thing. That just leaves answer (e) as the false answer. This is something you can test using data. Just go and grab some returns data with no missing observations, and estimate each.
- Q113 See answer to Q63 and also see the spreadsheet
Q63-Q113-20200610-REVISED.xlsx.
(hit F9 a few times to watch the simulation).
- Q127 The short answer is: multiply the t-stat for the mean by (1-rho) where rho is the auto-correlation. It is discussed on p. 107 of FFSI, in the middle of Section 1.3.17 ("How Robust is the t-Statistic for the Mean?").
The longer answer is: after the lengthy FFSI Section 1.3.16 on building a t-test to test the mean based on the assumptions that the data are distributed normality, independent and identically distributed, the following Section 1.3.17 asks, one at a time, what if these assumptions do not hold?
On p. 106/107 it says that if the only problem is a breach of dependence in the form of auto-correlation rho not being zero, then I published a paper in 2010 with a (now former) hedge fund manger (Dr. Olivier Ledoit) that shows that you just multiple the t-stat by (1-rho).
In this particular case t=2.2, rho=-0.15, so adjusted t = t*(1-rho)=2.2*(1-(-0.15))=2.2*1.15=2.53, which is even more significant.
There is a deeper (and slightly difficult) discussion of intuition in the middle of pp. 106-107 that argues that the usual t-stat has a standard error (in the denominator) that assumes that the variance of the sum is the sum of the variances. In the case of negative auto-correlation, however, a whole bunch of negative cross-product terms arise that are being ignored in the usual standard error calculation (I see a rho term appearing in the step after the asterisk on p. 106 in a simple two-observation case). So, the genuine standard error (accounting for the rho terms) is smaller than what the usual calculation would give (which assumes that these cross-product terms are zero). So, we need to inflate the t-statistic in this case. 30 pages of PhD-level algebra later, it turns out that just multiplying the t-stat by (1-rho) does the job.
- Q119 Please see the spreadsheet
http://www.foundationsforscientificinvesting.com/normal-pdf-discretization-2021.xlsx.
- Q130 A student asked "How are we able to calculate the t-stat when we don’t have information on the standard deviation of the data? Do you simply use Mean/(sqrt(1/n))?" The answer is twofold. First, no, that sqrt(1/n) is an estimate of the standard error for the correlation coefficient in the case where N is large and correlation rho is small (Equation 1.44 in FFSI; see Q10 above). Second, you are supposed to know the standard deviation of these data because the number is roughly the same for broad market indices in the US, UK, NZ, Japan, Germany, Australia, etc. This missing number is the number you always have in your head when you look at the daily return on a broad market index, to judge whether today's move is significant or not. It is 1%, as discussed in the second sentence of Section 1.3.11 on kurtosis. See Q52 and Q256 which use the same knowledge.
- Q134/2020MTQ22 This is based on pp. 80-83 of FFSI. This is a simplified version of the more complicated Q63 and Q113 in the Q&A book. See the spreadsheet I built to go with the solutions to Q63 and Q113: Q63-Q113-20200610-REVISED.xlsx
and hit F9 a few times to watch the simulation. The spreadsheet is also a wonderful opportunity to see the Merton Brownian motion random walk process in action: I used Equation 2.2 (discrete-time geometric Brownian motion RW) to generate the continuously compounded returns (CCR) r(n)=mu*tau+sigma*sqrt(tau)*z(n) and then I used P(n+1)=P(n)*exp(r(n)) to generate my simulated prices. With correlation questions like this, always go back to basics. The correlation is the covariance divided by a product of standard deviations, like in Equation 1.41 on p. 93 of FFSI. So, the correlation takes its sign from the covariance in the numerator. So, it comes down to the sign of the covariance. Now go back to basics again. The covariance is given by Equation 1.36 on p. 84 of FFSI. It is a product of moments: E[(X-mean)(Y-mean)]. So, the sign of the covariance is determined by whether the two terms (X-mean) and (Y-mean) have the same sign or different sign. If both X and Y tend to be above their means at the same time, and below their means at the same time, then you get positive*positive and negative*negative, both of which yield a positive covariance and thus a strong positive correlation. If, however, X tends to be above its mean when Y is below its mean, and vice versa, then you get positive*negative and negative*positive, both of which yield a negative covariance and thus a strong negative correlation. In 2020MTQ22, I told my students that FPH rose in price slowly and steadily by 34% and FBU fell slowly and steadily by 33%. Qualitatively, the plot must look like the red and the green plots in my spreadsheet simulation. The prices must be strongly negatively correlated, because in the first half (more or less) of the plot {P(FPH)-mean[P(FPH)]} is negative but {P(FBU)-mean[P(FBU)]} is positive. In the second half (more of less) of the plot {P(FPH)-mean[P(FPH)]} is positive but {P(FBU)-mean[P(FBU)]} is negative. So, in the first half of the plot the product of moments is mostly negative, and in the second half of the plot, the product of moments is almost mostly negative. There is only one answer here with a strong negative correlation: Answer(a). Unlike the questions in the Q&A book, I did not give enough other information here to deduce the correlation of returns, but you do not need it to pinpoint an answer.
- Q138/2020MTQ10 You should not be taking any analytical derivatives. You may have practiced calculating numerical derivatives using a given table of values. It was done that way in FFSI (pp. 33-35), and in several questions in the Q&A Book (e.g., Q6, Q12). There is, however, no table here. That's OK, because all you need is two values of the function, evaluated very close together. Those values could come from a table. Without a table, you can use the given function to build your own table. Of course, you only need two rows in the table, let's say at x=10, and x=10.001. So, let me find
slope=[f(x+h)-f(x)]/h
= [f(10.001)-f(10.0)]/0.001
= [log(sqrt(10.001)*e^{sqrt(10.001)})- log(sqrt(10)*e^{sqrt(10)}) ]/0.001
=[4.3137783-4.3135702]/0.001=0.208105, answer (f).
The step size h does not have to be 0.001, it just seemed like a good small number in this case. For example, you could try 0.01 or 0.0001, and the answer should still be about the same. That formula slope = [f(x+h)-f(x)]/h is the definition of slope. (Well, technically, the definition is to use this formula and let h go to an infinitesimally small value, but we are just approximating this with a small h.)
When you worked on Q3.2.2 (Active Alpha Optimization; p. 254 Q&A Book), Excel's Solver was doing exactly this. It was calculating changes in the value of the objective function for a small step in each of the 21 choice variables, and then moving your choice variables in a direction that was up hill, but subject to the constraints.
- Q140/2020MTQ5 This follows on from our Chapter 1 Section 1.3.13 true diversification discussion. One student asked if it is "none of the above," then what we should add to a stock portfolio to obtain true diversification benefits? Several ideas come to mind: a small holding in high-yield bonds via a high-yield bond fund (also known as junk bonds; they have lower creditworthiness and higher yields than investment-grade bonds, and can be more stock like than investment grade bonds); commercial real estate (either directly or through an investment fund); residential real estate (either directly or through an investment fund); exposure to some commodities (e.g., there is evidence that a small gold exposure is risky enough to diversify a stock portfolio and improve Sharpe ratios); some would say a small cryptocurrency holding, but I think this is not sensible; ownership in a private company (either directly, or via a fund); a small hedge-fund exposure (perhaps through a fund of funds); ownership of your own small business, etc.
- Q142/2020MTQ9 The CAPM equation is given: E(R)=RF+beta*[E(RM)-RF]. We need to put in the inputs and see which range of possible answers the E(R) number we get falls into. Assume at first that I do not recall the actual precise numbers. Before I do anything, my gut instinct is that the long-term rate of the return to the market portfolio (using equity only) is something like 9% or 10%. That is what I would get if my stock had a beta of 1. So, if I have a stock with a beta of 1.2, I expect a slightly higher number. That extra .2 in the beta is applied only to the market risk premium, so now I am tilting towards Answer (c), because I think that adds only about 1% to my previous 9% or 10% number. Now let me use the actual numbers (p. 7 of FFSI): RF=0.053, MRP=4.53%, CAPM yields 10.736%. These all put the answer in the range in Answer (c).
- Q148/2020MTQ33 Follow the hint! Look at the equation for beta and the equation for rho (i.e., correlation). They differ in only one term. All you need is std(x)=std(y), or approximately so to get beta=rho. In cases (a) and (b) these two equations give basically the same answer, so beta = rho, more or less, in both cases.
- Q150/2020MTQ26 I often asked my students to execute exactly this test. The statistic STAT = Zskew2 + Zkurt2 is the sum of two independent standard normal random variables (each is described on p. 58 of FFSI). So, by the definition of a chi-squared random variable (e.g., p. 59 of FFSI) it must be distributed chi-squared with 2 degrees of freedom. So, I am leaning towards (a), (b), or (c) already. These Z-stats are zero when the data are normally distributed, and non-zero otherwise. Because both Z stats are squared, the only way to get a rejection is if the statistic falls into the upper tail of the distribution (chi-squared tests typically use only the upper tail, even if the alternative hypothesis is two-sided). So, it must be answer (b). You can use the Excel sheet (MONTE-CARLO-EXERCISES-2020.xlsx) to simulate a chi-square with 2 degrees of freedom, so that you could see roughly where the upper critical 5% value should be (it is at about STAT=6).
- Q154/2020MTQ13 is about non-normality of returns. Discussed in great detail pp. 68-73 of FFSI. There are too many tail events and too many calm days for the distribution to be normally distributed.
- Q158/2020MTQ24 The form of this F-test is simply a ratio of sample variances. Students are often mistakenly tempted to put "N1" and "N2" into the test statistic. This error comes from a lack of understanding of the underlying intuition. This F-test is described in the box on p. 103 of FFSI. It builds upon the intuition we discussed for the chi-squared distribution (leading into the derivation of the t-statistic) and for the F-distribution. In 35+ years of reading stats books, I have not found a simpler explanation than this one.
I do find that it helps to look at simulations in spreadsheets. For example, these simulations for the chi-squared and t-distribution: MONTE-CARLO-EXERCISES-2020.xlsx. Can you amend my sheet to do the same for an F-distribution?
So, if these arguments do not help, then perhaps you just need to remember that the F-test for differences in dispersion is just the ratio of the sample variances. Nevertheless, please do ask if anything in particular is unclear. I will do my best to improve it.
- Q159/2020MTQ25 Answers (c) and (d) are the two scenarios under which the t-stat of the mean is invalid. The question asks when the traditional t-statistic for the mean is invalid. Note that in case (c) the traditional t-statistic for the mean is invalid because of dependence. (Yes, in case (c) you can adjust it as described on p. 107 of FFSI, but then you no longer have the traditional t-statistic for the mean.)
- Q161/Q133 (see also Q425) In this question, the T-costs are the (ask price - mid-spread price)*quantity + commission. So, that is ($6.34-$6.295)*100 + $30 = $34.5. Let me add some additional details: The total cost of the stock, including all T-costs, is (ask price)*quantity + commission = $6.34*100 + $30=$634 + $30 = $664. The fair value of the stock, however, was only (mid-spread price)*quantity = $6.295*100 = $629.50, where $6.295 is the mid-spread price. The difference between these two numbers is the answer to the question asked.
[Follow up response to further questions: If the ask price is $6.34, and you buy 100 shares, then you pay $6.34, and that includes the T-cost of the half-spread above fair value. The half-spread cost that built into this stock price is unavoidable. The $6.34 price tag of the stock includes the underlying T-cost of the spread that results from the way the NZX does business.
In the morning and the afternoon, the bid-ask spreads tend to be wider, even if fair value does not change.
The $6.34 price tag does not, however, include the commission you pay to your broker to let you bring your trade to the NZX.
Analogy Suppose the fair value of a can of baked beans is $1. If you buy the beans at the supermarket, maybe the price tag is $1.20. If you buy the beans at the corner dairy, however, then maybe the price tag is $1.50. The fair value of the beans is the same in both cases, but if you buy the beans at the corner dairy, then they pass their higher T-costs on to you in the form of a higher price tag. If you have to take the bus to go to the store to buy the beans, then the price of the bus ticket is added to your T-costs of buying groceries, but it is not displayed on the price tag of the beans in the store.
The $1 fair value of the beans is like the mid-spread value. The $1.20 price is like a middle-of the day ask price. The $1.50 price is a like an early morning or late afternoon ask price. The bus ticket price is like the commission.]
- Q162/Q134 This is one of my favorite parts of the course because it is both very simple to explain and very applied to you in your personal investing. Let R be the return before fees. Let e be the expense ratio (that is, the fee) per annum. So R=0.0800 and e=0.0005 here. Then the net return on the fund is R-e=0.0795, because the fee is subtracted each year.
Then without the fee (i.e., gross): FV=$10,000*(1+R)^N=10000*1.0800^10=$21,589.25
With the fee (i.e., net): FV=$10,000*(1+R-e)^N=10000*1.0795^10=21,489.508
The difference is about $100, answer (c).
Jack Bogle loved this sort of calculation because now try it with e=1.25%, the typical fee for an actively managed fund. It is a simple argument for buying low-fee funds, as discussed in Section 2.4.7.
- Q167/Q139 We often focus on KiwiSaver rules and regulations.
- Q168/Q140 Let me reassure you first that I am not going to ask you any exam questions this year about stock splits. There has been a dramatic reduction in their use in the last five to ten years. This must be an old question. A stock split is a simple idea. Suppose you bought a $10 share of stock and the company did really well and the stock price went to $30 a share. The company might declare a "three-for-one" stock split. They take back your $30 share and give you three newly issued ones worth $10 each. Economically, you have the same value stock ($30) but the count of shares you have increased. Let us work it out.
Assume that you buy one share at the ask on Monday (1080). It looks like four-for-one split took place (which you correctly guessed). This means that walking into Friday you have 4 shares. Each share pays you a dividend of 25, and can be sold for 225 (the bid on Friday).
So, return = (final-initial+dividends)/initial = (4*225-1080+4*25)/1080=-80/1080=-7.4%, answer (c).
I think you will see the logic. I am guessing that you did not collect 4 dividends when you did it. Easy to miss.
- Q172/Q144 (see also Q215/Q187, Q310/Q282) Each of answers (a), (b), and (c) is a correct statement, but (c) is not relevant to the question (Answer (c) might as well say that "the sky is blue on a sunny day"; true, but not relevant here).
- Answer (a) is correct, because most investors realize that in order to build wealth over the long run, they must take exposure to risky assets like stocks. So, they are resigned to the fact that they must be in stocks. That's an unchanging given, and so is not part of our decision making process. That's why we can remove benchmark return and risk from our objective function. After that, we ask the question "Do they want us, as portfolio managers, to step away from that benchmark?" Our clients are much more fearful of us doing this, using stock selection or benchmark timing, than they are of us just passively following the benchmark. Ultimately the answer is that we take on active risk only if the active return outweighs the risk taken, and we have the client's permission to do so.
- Answer (b) is really just a mathematical expression of the same statement made in Answer (a). That is, Answer (a) and Answer (b) are saying the same thing, and they are the reason why we can remove benchmark return and risk from our objective function.
- Answer (c) is about whether, when an active portfolio manager steps away from the benchmark, they should engage in benchmark timing. The statement is correct (most institutional asset mangers, that is asset managers managing money on behalf of other institutions, do not engage in benchmark timing), but Answer (c) is about stepping away from the benchmark, while the question is about the underlying benchmark itself. So, Answer (c), although a correct statement, does not answer the questions. Note that there are many asset managers who engage in benchmark timing, but for asset managers managing money for other institutions it is a minority.
- Thus, Answer (e) is correct.
- Q173/Q145As discussed in FFSI on p. 312, you need T>=N for the VCV to be invertible. Three months of data is about T=3*21=63 observations. If N=100 stock, then the VCV is not invertible.
- Q175/Q147 FLAM says IR=IC*SQRT(BREADTH). If you multiply the BREADTH by 2, then, because the BREADTH appears under the square-root sign, the IR increases by a multiplicative factor of SQRT(2). So, IR increases by about 40%.
- Q178/Q150The consequences of a margin trade going against you appear in Sections 2.4.5 and 4.3.2 of FFSI.
- Q179/Q151 Betas relative to T are perfectly related to returns, like in the CAPM. So, if the stock returns (for TEL, FPH, PPG) are less than RF, then they must have negative betas, like in the CAPM.
- Q181/Q153 Answer (c) is not correct because empirical evidence I have seen suggests that on small U.S. stock orders, ECNs and the NYSE have roughly the same costs, and in large orders, the NYSE has a cost advantage. This topic needs to be revised in the next edition.
- Q182/Q154 Dividend time lines appear in Section 2.5.1 of FFSI.
- Q183/Q155 It is a market order to buy 100,000 shares. So, the first thing to do is look and see if anyone is offering that many shares. Yes, I see 75,000 offered at 387, 10,000 at 388, 10,000 and 389, and we will need 5,000 out of those offered at 390 (all in cents per share). So, total cost is the sum product of those: (75,000*$3.87)+(10,000*$3.88)+(10,000*$3.89)+(5,000*$3.90)=$387,450. There were 100,000 shares purchases, so the average price is $387,450/100,000=$387.45 or 387.45 cents per share. We usually quote everything in dollars now, but the principle is the same.
- Q185/Q157 This is about order precedence.
In (a), the limit order will sit in line behind the 75,000 shares already there, whereas the market order is immediately executable, so that is false.
In (b), the market order is immediately executable and the limit order will wait in line, so that is true.
In (c), the limit order is marketable, like the market order. So that is true.
Hence answer (e) is correct.
It is not that market orders are executed more quickly than limit orders, but that marketable limit orders and market orders (assuming sufficient depth) are both immediately executable, and limit orders that arrive and have to wait in line behind other orders are not immediately executable.
- Q186/Q158 Fund fees appear Section 2.4.7.
- Q187/Q159If you sold an option on one share, then you trade delta shares of stock to hedge it. If you sold an option on N shares, then you trade N*delta shares of stock to hedge it. If delta is positive, then you buy stock to hedge. If delta is negative, then you sell stock to hedge.
- Q188/Q160 We have not dealt with this exactly, but we have dealt with many parts:
You are buying, so you hit the ask: $0.95.
This is a quote per share. The contract covers 100 shares. So the option price is $95 for one contract.
The commission is a flat $10 plus a $0.75 per contract fee. You buy one contract only, so you pay $10.75 commission. Add that to the option price to get $105.75.
OK? We have not done protective puts, though, arguably, we should have.
- Q191/Q163 Let me use simple net return (SNR) =(final-initial+dividend)/initial.
SNR(1)=(430-455+30)/455=5/455=0.010989
SNR(2)=(425-430+0)/430=-5/430=-0.016279, giving answer (a). The important part is knowing where the dividend goes. This has not been emphasized in this context, but the SNR formula is one you should know.
- Q194/Q166 From Q3.2.2 (Active Alpha Optimization; p. 254 Q&A Book), the key is that the IR=alpha/omega, and the omega appears in the middle term of the objective function Equation 2.81 p. 290. That is, omega^2=sigmaP^2-sigmaB^2. So, you need IR=alpha/omega, where alpha=0.04300 is given to you, and omega^2=sigmaP^2-sigmaB^2 = 0.1240^2-0.1063^2. So, IR=alpha/omega=0.04300/[sqrt{ 0.1240^2-0.1063^2 }] = 0.04300/0.0638459 = 0.6734957, answer (c). In this question you were supposed to spot that the entire setup looked like Q3.2.2 (Active Alpha Optimization; p. 254 Q&A Book): I gave you sigmaB and I gave you sigmaP and I told you that beta=1 (which means that omega^2=sigmaP^2-sigmaB^2) and you are calculating an IR like in Q3.2.2 (Active Alpha Optimization; p. 254 Q&A Book). So, there were lots of cues and clues.
- Q203/Q175If you are uninformed, then the price rise is temporary only, and these higher prices are T-costs, and they are unambiguously bad for you (answer (b)). This higher price will be recorded as a transaction price that is disseminated online, but there is no information in it. In a liquid stock, the order book on the ask side will fill back in again in a minute or two. If you think this price rise is good for you, then you have to ask what is the next step? If the next step is to sell your stock, then at what price can you sell it? The answer is that it will be at the bid price, and if the order is large enough, price impact when you sell means that you will walk into the CLOB and sell some of your stock at prices even worse than the bid price. This means that every price you sell (from the bid price down) is lower than every price you bought at (from the ask price up). This is not good for you. When I mark to market (that is, when I value my stock holdings), I always use the bid price. Even that may be optimistic, given possible market impact. Your trade did not move the bid price, so you did not change the price you can sell your stock at, so there is nothing good in this price impact.
- Q207/Q179This is the FLAM IR=IC*sqrt(BR). We mentioned this on p. 304 of FFSI. BR is breadth = number of stocks you follow * number of independent forecasts of return you make on them per annum. If we multiply BR by 1/2 (by halving the count of forecasts), then that half gets square rooted, and sqrt(1/2)=0.7071 approx 0.71. Conversely, if we doubled the breadth, then the BR would go up by a factor of 2, and so the IR would go up by a factor of sqrt(2). Do you see? Ask again if not.
- Q208/Q180 The correct answer is that the standard error of the estimator of the mean is unchanged.
- Q211/Q183 (see also Q232/Q204) Active anything (return, portfolio weights, risk, beta) is always portfolio quantity less benchmark quantity:
active return=RP-RB
active weights=hP-hB
active risk=sigmaP^2-sigmaB^2
active beta=betaP-betaB=betaP-1
So, it is answer (e), none of the above, because the two correct answers are not given together.
- Q214/Q186 IR=alpha/omega, where omega is active risk. She needs to generate enough alpha to deduct expenses and fees of 100 bps and still have 50 bps left over for the client. So, she needs an alpha of 150 bps. With an IR of 0.60, she needs an omega of 250 bps. Think of this as walking up the budget constraint in Figure 2.17 (p. 296) until the omega is high enough to generate a target alpha.
- Q215/Q187 (See also Q172/Q144 and Q310/Q282 on similar topics). Each of answers (a), (b), and (c) is a factually correct statement, but only answer (a) answers the question posed. The question is about benchmark return and risk, and why we dropped them from our objective function (see bottom p. 290 of FFSI). Answer (b) is, however, about benchmark timing, that is stepping away from the benchmark with a beta that is not equal to 1. Answer (c) is about aversion to active risk, that is, aversion to the risk engendered by stepping away from the benchmark. Whether this aversion is zero, low, medium, or high, we will still drop the benchmark return and risk terms from the objective function because they are not a function of our choice variables. Note that I don't think we used the phrase "maverick risk" this year, but it is defined in the question and the basic idea should be clear.
[Additional deeper details on the comparison of Q172/Q144 and Q215/Q187 which you do not need to read unless you are very interested: In Q172/Q144, answer (a) says that investors are resigned to facing the risk of the benchmark. That is, investors have accepted that although they fear benchmark risk, they must expose themselves to it over the long run, in order to earn the long-run equity market risk premium, in order to build wealth. As a consequence, we, as buy-side portfolio managers, have already won that sales pitch. Even Jack Bogle made that sales pitch successfully, and he was pushing passively managed funds and he was dead set against active portfolio management. So, the investors are already on the "invest in the stock market" roller coaster ride, and we can drop that term from our objective function. The remaining terms are concerned with active positions: benchmark timing and stock selection.
Now think about that active management. Well, in Q215/Q187, answer (c) says that investors fear maverick risk more than they fear benchmark risk. Yes, that is true. Many investors have no faith in our ability as fund managers to pick stocks or time the market. So, they have a much higher aversion to active risk than they do to passive benchmark risk. This is going to be a tougher sales pitch. So, in building those other components of our objective function, we need to use a higher risk aversion coefficient than we used for benchmark risk. You see Equation (2.75)? It has three lambdas in it. The fear of maverick risk means that lambdaBT (aversion to benchmark timing risk) and lambdaR (aversion to stock selection risk) are each higher than lambdaB (aversion to benchmark risk). So, this "maverick risk" aversion statement is a statement about the relative size of those three lambdas, not about whether that first benchmark return and risk term belongs in our ultimate objective function. If you really want to, you can leave the first term in Equation 2.75 (kappaB-lambdaB*sigmaB^2) in the objective function. You do not have to drop it off. It is just that it is not a function of our choice variables, so it is easier to drop it off. Whether it is there or not, however, those lambda risk aversion terms have to have the relative sizes mentioned, and that is what this maverick risk statement is about. It is not about the ability to discard the first term.
End of extra details.]
- Q219/Q191 For telephone orders, the broker charges 70 bps (0.70%) with a minimum fee of $35. It is a$12,000 trade, so the commission is $12,000*0.007=$84. That is above the minimum of $35.
- Q220/Q192 For internet orders, the broker charges 30 bps with a minimum of $30 commissions. 10,000 shares at $2.20 costs $22,000. $22,000 times 0.003 is $66. When I sell 1000 shares at $2.10 the value is only $2,100. Then $2,100x0.003 is only $6.30, but they say the minimum commission is $30. So they will charge me $30. This is an actual commission schedule from ASB Securities in NZ. So, the total is $96 commission.
- Q221/Q193 This goes back to discussion of passive long-term investing in Chapter 1. Remember we said that if you could 1% per annum to your investment over the 40 or 50 years until retirement (assuming you are 21), you add about half as much wealth again at retirement (i.e., +50% wealth)? Well 0.5% extra adds about 20%. You don't have to memorize these. Just ask, what if I used 9.5% instead of 9% to compound for 40 years. Let's test it with $1000. $1000*(1.09^40) is $31,409 what about at 9.5%?
Well, $1000*(1.095^40) is $37,719. Divide the second one by the first, and it is 20% bigger. Where did 9% come from? I made it up. Try 7% or 8% and it is almost the same ratio.
- Q226/Q198 On p. 190 of FFSI, a paragraph discusses P/CF ratios. I always mentally invert it to get CF/P. Then that looks like a return on investment. In my head I have 10% as the long-run return on the market. So, CF/P=10% is fair, higher is attractive, lower is unattractive, other things being equal. ...but then you have to invert back into P/CF. So, P/CF=10 is fair, P/CF lower than 10 is attractive, P/CF higher than 10 is unattractive, other things being equal. We have P/CF=2.99 here, so that's a CF/P=33%, which is great! Answer (a).
- Q227/Q199 From Q226/Q198 we already argued that this stock has an attractive P/CF ratio. For P/E, Lynch and Benjamin Graham say that lower is better, other things being equal. So, look at the column comparing P/E of the target firm with its peers. It has the lowest of the group, which makes it attractive. In practice, we worry about different gearing, but you are explicitly told to ignore that. I have to say that I would like to compare the P/E to the forecast growth rate in earnings per share of the same company (see p. 190 of FFSI), but the growth rate in EPS is not reported on this screen. (Bloomberg lets you edit this screen and add whatever you want! I just printed the default screen.)
- Q229/Q201 Like Q226/Q198 and Q227/Q199, let me invert the P/CF to get CF/P=1/4.85=21%. That's very attractive, other things being equal. Like Q226/Q198 and Q227/Q199, let me compare the P/E to the P/Es of the Bloomberg peers (do watch out for that in practice, sometimes the "peers" are very odd choices). Based on that list, the P/E of 38 looks unattractive. Answer (b).
- Q230/Q202 You really need to have completed the active alpha optimization exercise before doing this. The key is that the IR=alpha/omega, and you need the omega, and it appears in the middle term of the objective function for Q3.2.2 (Active Alpha Optimization; p. 254 Q&A Book): omega^2=sigma_P^2-sigma_B^2 (...and don't forget to square root it).
- Q232/Q204 (see also Q183) Active anything (return, portfolio weights, risk, beta) is always portfolio quantity less benchmark quantity:
active return=RP-RB
active weights=hP-hB
active risk=sigmaP^2-sigmaB^2
active beta=betaP-betaB=betaP-1
If active beta=0.5, then betaP-betaB=0.5. But betaB=1. So, betaP-1=0.5. So, betaP=1.5 which is quite aggressive. Answer (a). ASIDE: I rather suspect that in a long-only implementation, you would not be able to run your Q3.2.2 (Active Alpha Optimization; p. 254 Q&A Book) with the constraint beta=1.5 because it is too aggressive. Yes, I just tried it. I got to 1.34 and it conked out. You cannot squeeze enough juice out of the betas to get it that high in a long-only implementation. If I allow the fund manager to go short, however, I should be able to short some low-beta stocks and over-invest in high-beta stocks and get beta=1.5. Yes, I increased T/O to 200% and allowed up to -10% weights, and I got a beta of 1.5 by shorting low-beta stocks.
- Q233/Q205 Like Q226/Q198 and Q227/Q199 and Q229/Q201, let me invert the P/CF to get CF/P=1/2.50=40%. Wow. That's great, other things being equal. Answer (a). ASIDE: As always, this is just a single signal among a ton of data. This is the high in this stock over the last 10 years, and it dropped from $52.98 to $6.35 during the COVID-19 market crisis of 2020. It has bounced back to nearly $30 a share mid-2020, up 350% since the low of mid-March, considerably outpacing the S&P500 which is up 40% by mid-2020. Avis's competitor Hertz filed for bankruptcy mid-2020.
- Q235/Q207 After using relative spreads in Q3.2.2 (Active Alpha Optimization; p. 254 Q&A Book) you will be expected to know the general size of relative spreads for large-cap NZX stocks: between about 50 and 100 bps in the Otago University FINC302 2020 dataset.
- Q236/Q208 This is about the PEG ratio discussed on p. 190. If P/E were 6 and forecast growth rate in EPS were 6%, then Lynch would say this is fairly priced. The P/E is much higher here (because the price is much higher). So, this stock is overpriced and unattractive by this simple rule.
- Q238/Q210Someone asked what does 'the stock closed down 5 pennies on this day' mean? One penny = $0.01. It is also called 1 cent. The little legend on the plot shows that the last price on this day was $1.88 (I can see that at the far right also), and the closing price on the previous day was $1.93. So, yes, the stock did "close down 5 pennies". This means the closing price was lower, by $0.05, compared with the previous closing price. It closed (i.e., ended the day) down $0.05 compared with the previous day. So, it closed down $0.05.
- Q239/Q211 The odd one out is answer (c) because the active alpha optimization is a very conservative technique. When you run it on Q3.2.2 (Active Alpha Optimization; p. 254 Q&A Book) you should find that the optimal portfolio alpha is somewhere in the region of 1%-5%, and typically in the middle of that range. Yes, when we remove constraints and let it sell short or allow more turnover, we might get an alpha if 10%, say, but not with standard risk aversion and the many constraints you will implement in Q3.2.2. This answer for RTAA (the Active Alpha objective function Equation 2.81) is basically 5 or 10 times too big.
- Q240/Q212: I am looking to see whether RTAA is less than alpha (it has to be because RTAA (Equation 2.78 in FFSI) = alpha-risk penalty- T-cost penalty. So RTAA must be less than alpha. That fails on (c), so (c) is not valid. Oh, (d) cannot be valid either because you cannot get a negative alpha in an optimization. That's because (as you will see in Q3.2.2 [Active Alpha Optimization; p. 254 Q&A Book]) doing nothing, and just holding the benchmark gives an alpha of zero, which is superior to a negative alpha. In an maximization, you would never go from zero to a negative. This will become clearer in Q3.2.2.
- Q244/Q216 The only thing a little different from other exercises here is that you had to read the delta of 99.98% (which is 0.9998) off of a Bloomberg screen. In this case, the investment bank has sold this call option to a corporate client. So, the corporate client is long the option, and the investment bank (acting as a market maker) is short the option. In order to hedge their exposure, the bank must replicate a long call option position. They need to buy delta shares of stock for each share covered by the option. So, they need to buy N*delta = 1,000*0.9998 shares. This is 999.8 shares, which they will just round to 1,000 shares. So, the bank must go long 1,000 shares to hedge. Note that if the bank were to instead go short the 1,000 shares, then they would have compounded the risk, not hedged it, because then they would have two positions that go against them if the stock price rises, instead of having offsetting positions.
- Q246/Q218 Answer (d) sounds like a market-neutral fund; it is like the long-short fund I set up in my brokerage account.
- Q247/Q219Note that 1.5019 is the USD price of one GBP. This question was built for me by a US investment banker (markets, not corporate finance). This is exactly how he phrased it. This is a very interesting question. Q247/Q219 uses the Bachelier formula, which you have seen mentioned in Section 3.2 and in Figure A.2 (recall it is an approximation to Black-Scholes). You should use an approximation to Black-Scholes pricing. The Bachelier formula is the only approximation to Black-Scholes pricing that we have seen. It says that c=0.4*S*sigma*sqrt{tau}, where tau is time to maturity in years. In this case, I can let S be the price per GBP and multiply by the number of GBP. I get c = 0.4*S*sigma*sqrt{tau} = 0.4*$1.5019*0.13834*sqrt{0.5} = $0.058767 (i.e., the price of an option to buy 1 GBP). So, I multiply this answer by the number of GBP involved (6,500,000) to get $381,986, answer (e). If you used the 1/sqrt{2*pi} in the original Bachelier formula, instead of just setting that equal to 0.4, you would get a very slightly different answer, but (e) would still be the best choice.
- Q253/Q225 See the extended answer to Q506/2020MTQ19.
- Q255/Q227 I provide 30% of the purchase price (that is my initial investment). My broker lends me the other 70%. I buy 1,000 shares at 375 cents per share. Total cost $3,750. My investment is 30% of this: $1,125. I owe $2,625 to my broker. I will lose 100% of my initial investment if the value of my stock (currently $3,750) falls until there is only just enough left to pay back the broker. So, if my 1,000 shares drop to be worth only $2,625. That is, $2.625 per share. Rounding to pennies, that would be 262 per share, answer (d).
- Q258/Q230 Do not confuse "The spot exchange rate was observed to be 1.48291/1.48299 USD/EUR" with "The quoted FX rate was EUR/USD 1.48291/1.48299". The former is to be read literally; the latter uses the standard FX quoting convention. Don't worry, any exam question will be clearer than this. This will be rephrased in the next edition.
- Q259/Q231 See Q506/2020MTQ19.
- Q262/Q234 Someone asked "when we send in a market or limit order to buy but at the price of bid, will our order be executed? for example, in option a) I submit a limit order to sell at a price of $4.42 but who will be buying my stock? Does it still go through the buyers side of the CLOB? Also why would my order be executed first?"
[Answer to student question You asked about submitting a market order or limit order to buy at the bid. Part of your question does not make sense. I will explain in a moment. You also asked who is buying if I submit a limit order to sell at $4.42. I will explain in a moment. I need to walk you through the thinking, step by step.
First, if I send in a market order to buy, then I do not state a price. Market orders name the stock (ABA in this case) and the quantity (e.g., 100 shares), but never a price. In some places a market order is called an "at market" order. This is more accurate. You are trading at whatever the market price is, but you are not choosing the price. You are a price taker, not a price maker.
A market order to buy will hit the best ask price if there is enough depth there. For example, a market order to buy 100 shares using Table 1.17 (ABA) will be executed at $4.45. Then the top line on the right-hand side of the CLOB will change to say "$4.450 3,479 1" after my order is executed. My market order to buy is simply taking the prices as given.
If I instead send in a limit order to buy 100 shares, however, then I must specify the limit price. Every limit order names the stock (ABA in this case) and the quantity (e.g., 100 shares), and the limit price. Then I am telling my broker that I want to buy 100 shares at this price or lower. I can choose any price I want. For example, I can submit a limit order to buy at $4.00, or $4.01, or $4.02, or $4.03, ....., or $4.40, or $4.41, or $4.42, or $4.43, or $4.44, or $4.45, or $4.46, or $4.47, ...., or $4.60, or $4.61, ....etc. You can put any price you want on that limit order. You can put the bid price, the ask price, or any price!
Ask yourself this question: In Table 1.17 the top line on the left-hand side says "2 3,090 $4.410". How did that line appear there? Maybe it appeared there because one traded submitted a limit order to buy 1,000 shares for $4.410, and another trader submitted a limit order to buy 2,090 shares for $4.410. I cannot tell whether it was 1,000 and 2,090, but it was two orders that add to 3,090.
There is nothing to stop you submitted another limit order to buy 100 shares at $4.410. If you submit a limit order to buy 100 shares at $4.410, then the top line on the left-hand side of the book will change to say "3 3,190 $4.410". That is, three traders (i.e., two others plus you) are now bidding for stock at $4.410 per share.
If you do submit a limit order to buy at 100 shares at $4.410, however, you have to wait in line behind the other two traders, because they got there first. That is called time priority. So, for example, if you submit a limit order to buy 100 shares at $.410, and if another customer then sends a market order to sell 3,000 shares, you will not get an execution. That is, first come first served at any limit price means that you will still be waiting in line. So, after that market order to sell 3,000 shares, then the top line on the left-hand side of the book will change to say "2 190 $4.410" and one of those traders is you still waiting to buy your 100 shares.
If I submit a limit order to sell 100 shares at $4.42, then I am offering a more attractive price than any other trader. So, by price priority, I jump to the top of the offer side of the book. Then the top line on the right-hand side of the book will change to say "$4.420 100 1", and that is my order. This also gives a lower bid-ask spread of only one penny. As soon as another trader submits a market order to buy stock, it will hit my limit order to sell. I am making a market at this price. I am a price maker, not a price taker.
END of answer to student question.]
- Q264/Q236 Someone asked why it is not answer (b). Answer (b) is false because what if the limit price is $4.41, or any lower price? Such an order is immediately marketable, with no risk of non-execution.
- Q267/Q239 uses the Fundamental Law of Active Management! Remember IR approx= IC*sqrt(BR). IC=2% and BR=225*4=900.
- Q268/Q240 (see also Q426) Before doing any calculation, my gut instinct is that it must be a number close to 30% ((c) or (d)). In Chapter 1, we said that for a lump sum investor, an extra 1% increases final wealth for you folks (say 45 years to retirement) by 50%, and for an annuity investor, an extra 1% increases final wealth for you folks by about 30%.
Both have the same growth rate g=4%. 1. Draw a timeline. Actually, you need two timelines, one for 8% and one for 7%! 2. Write down algebra for FVGA8/FVGA7. 3. Cancel out the first cash flow (which is the same in each and cancels in this ratio). 4. Stick in numbers and calculate the ratio.
Here are the details: FVGA(C,R,g,N)=(C/(R-g))[(1+R)^N-(1+g)^N]. So, I need to take the ratio FVGA(C,8%,4%,43) over FVGA(C,7%,4%,43). Drop the C because it cancels. The numerator is (1/(.08-.04))*[1.08^43-1.04^43] and the denominator is (1/(.07-.04))*[1.07^43-1.04^43]. I get 549.1536/431.462=1.27277, a 27.3% increase.
- Q270/Q242 Unlike a market order, every limit order has a limit price associated with it. Some limit orders are immediately marketable (because a counterparty is already sitting there willing to trade your full quantity with you at your limit price), and some are partially executable (because a counterparty is already sitting there willing to trade part of your quantity with you at your limit price), and some rest in the CLOB until an opposing order arrives (because no counterparty is already sitting there willing to trade any part of your desired quantity with you at your limit price). In the last case, you might never get an execution if market prices move away from your limit price.
For example, this question asks about a limit order to sell 5000 shares. Consider some cases:
Case 1: Suppose you enter a limit price of $1.09 for your 5000 shares. Then you join the queue behind the two traders who already have limit orders to sell at $1.09, and in this case the top line on the right-hand side of the book will change to "$1.09 102,928 3". If bad news comes out about the company, market prices may fall away from your limit price, and you get no execution. This must have happened to many limit sell orders for AIR back in the first quarter, when AIR's stock price collapsed by about 75%. Alternatively, a market order to buy 120,000 shares might arrive next, and all your stock gets sold. ...but you just don't know.
Case 2: Suppose you enter a limit price of $1.07 for your 5000 shares. Well, there are 2 traders bidding $1.08 for 3,427 shares, and there are three traders bidding $1.07 for another $37,310. So, in this case, you will sell 3,427 shares for $1.08 (just above your limit price; remember that a limit order says "this price or better"), and you will sell the remaining 1,573 shares for $1.07. So, in this case, you limit order is immediately marketable. The top line on the left-hand side of the book will change to "N 35,737 $1.07" in this case, and I cannot tell from the data what N is.
The only good answer here is (e), because even if you know the limit price, there are cases where you do not know whether you will even get an execution.
- Q272/Q244 It is answer (e) because there are no bids. My market order is not marketable because nobody is bidding for this unloved stock.
- Q276/Q248 Answer (e), because it is diversification that explains the low risk in Portfolio B.
- Q278/Q250 Do not confuse "the exchange rate was 0.7296 USD/NZD" with "the quoted rate was NZD/USD 0.7296". The former is to be read literally; the latter uses the standard FX quoting convention. Don't worry, any exam question will be clearer than this. This will be rephrased in the next edition.
- Q279/Q251 This is about IR=alpha/omega. In this case, the alpha has to cover the fees and the active return. So, we need an alpha of 150 bps. You can deduce the omega, since IR=0.50 is given.
- Q280/Q252 is a little too detailed, because it is looking back at one year only out of a sample of years, rather than looking at your year of data that you actually worked with.
- Q281/Q253The key is that the area under the smooth curve must be 1. So, I estimated are by figuring area in a small rectangle and counting rectangles.
- Q284/Q256 The key is the 1% rule again. That is, standard deviation of daily returns to a broad market index is 1%, and about 95% of the area under the curve is therefore between +/- 2 standard deviations. Look at Figure 1.16 on p. 71 of FFSI. This is the actual correct picture. It is the same as the picture on Figure C, but it is easier to see in Figure 1.16 where the standard deviation appears. We know that
- 95% of the probability mass of a normal distribution lies between plus and minus 1.96 standard deviations. This is true for every normal distribution.
- 1.96 is very close to 2. So, let us just call it 2 standard deviations.
- The standard deviation of daily returns to a broadly diversified equity index in a developed country is about 1%.
- The smooth curve in the correct subplot is a normal distribution with the same standard deviation as the daily returns to the S&P500, which is 1%.
- So, 95% of the probability mass under the correct smooth curve must lie between plus and minus 2%.
- You can completely ignore the jagged curves and look only at the smooth curves. Find the one with 95% of the probability mass between plus and minus 2%.
- The only one that matches is answer (c).
- Q285/Q257 You can calculate it numerically using C(S) as [C(10.001)-C(10)]/0.001=[1.128492-1.1283792]/0.001=0.1128. One student asked why the delta is not 0.5. After all, this option is at the money. The short answer is that the Bachelier formula is really great for pricing at-the-money options, but it is terrible at giving you any information about hedging.
I created a spreadsheet abut Bachelier option pricing approximations Q257-Q320-20200610.xlsx for the curious.
- Q286/Q258 You can calculate this in your Q3.2.2 (Active Alpha Optimization; p. 254 Q&A Book) spreadsheet if you wish, but let me give a simple example. Suppose there are only three stocks. Suppose the benchmark weights are
hB=
0.20
0.40
0.40
Suppose that your portfolio is
hP=
0.25
0.35
0.40
So, you over-weighted stock 1 by 5% and underweighted stock 2 by 5%. Then your active weights are hP-hB. In fact, active anything is always that quantity for P less that quantity for B (true for weights, betas, risk). So, active weights are
hP-hB=
0.05
-0.05
0.00
So, active weights sum to zero. In fact, that's a nice opportunity to discuss turnover. Suppose you started off with benchmark weights (like in Q3.2.2 [Active Alpha Optimization; p. 254 Q&A Book] but using this 3-stock example here), and you had $100,000 invested, and you sold off $5,000 of stock 2 and used the proceeds to buy $5,000 of stock 1. Then you would go from portfolio weights hB to hP as above. Well, you did 5% one-sided turnover (i.e., counting only purchases, say), but 10% two-sided turnover (counting purchases and sales). So, abs(hP-hB)'i, where i is a vector of ones is (0.05 0.05 0.00) (1 1 1)'=0.10, which counts the two-sided turnover, like in the constraint in Q3.2.2 (Active Alpha Optimization; p. 254 Q&A Book).
- Q287/Q259The key is that the area under the smooth curve must be 1. So, I estimated are by figuring area in a small rectangle and counting rectangles.
- Q288/Q260 (see also Q320) This is another opportunity to test your Bachelier option pricing knowledge. This is from an actual job interview (it is from several job interviews in fact; it is quite common). The interviewer usually says something like "you have 10 seconds to give the quote". Well the only thing that changed is the time to maturity, which changed by a multiplicative factor of 0.5. That time to maturity term appears under a square root sign in the Bachelier formula. So, the option price changes by a multiplicative factor of sqrt(0.5). The answer is $12 * sqrt(0.5) = $8.49, answer (b).
In fact, I did it in my head much faster than this. I memorized sqrt(0.5)=0.7071 long before you were born, because I use it so much. So, in my head I already said that 7 times 12 is 84, and I need to move the decimal point over by one place, so the answer must be about $8.40, but I actually needed to multiply by 7.07, which is 1% larger, so in my head I am already at $8.484, which is 6/10ths of a penny from answer (b), and I am done before I even get the calculator in my hand.
- Q289/Q261 I expect you to have a feel for size of spreads with the sample of NZX stocks used in the long-answer questions.
- Q290/Q262 The short answer is that the figure shown is not the space in which we are conducting our optimization. So, there is no reason why P* should appear optimal there, which is answer (d). Here are more details. When we discussed our active alpha optimization routine, to be used in Q3.2.2 (Active Alpha Optimization; p. 254 Q&A Book), we said that we would be working in "active space," that is, we would be thinking about the optimality of our decisions only in terms of deviations from the benchmark. So, the figure shown is the wrong space for our optimization. We need E(RP-RB) on the vertical and we need STDEV(RP-RB) on the horizontal. That is, we need the mean and standard deviation of active returns, not of total returns, on the axes. When beta=1 (which you may recall imposing in Q3.2.2, E(RP-RB)=alpha, and STDEV(RP-RB)=omega. If you put these on the axis, you get Figure 2.17 on p. 296 of FFSI, and in that figure, P* is optimal.
- Q291/Q263 Like Q290/Q262, this is the wrong space for our optimization. So, it does not matter that T has a higher Sharpe ratio. Unlike Q290/Q262, now it comes down to details. We stepped through several candidate objective functions before arriving at the objective function you optimized in Q3.2.2 (Active Alpha Optimization; p. 254 Q&A Book). WE argued that if you compare total return to total risk, the end result is that your portfolio will be too aggressive. That is, your aversion to total risk is not high enough to suppress the active risk (i.e., the risk of stepping away from the benchmark), and if you compare total return to total risk, you will end up with a portfolio that is much too aggressive in terms of active risk. That is, you will step too far from the benchmark. It is also true, though not emphasized in 2020 that T will very likely involve short selling and also benchmark timing (both of which we avoided in our basic GKLO implementation). It is also true, though not emphasized in 2020 that T will have the same IR as P*. Answer (a) and answer (b) are correct, but there is a bit more detail in this question than you need this year.
- Q292/Q264 Answers (a) and (c) are correct. For (a) recall that T is obtained by optimizing in total return total risk space. So, it will be too aggressive in terms of active risk (as discussed on p. 298 of FFSI). For (b), we can see in Figure 2.17 of FFSI (p. 296) that T and P* are both on the budget constraint. They therefore have the same IR (i.e., the same Sharpe ratio in active space). For (c), I can see in Figure 1.18 (p. 121 of the Q&A Book) that the dashed frontier is the minimum-risk beta=1 frontier. So, there is nothing to the left of this with beta=1 relative to B. So, in particular T must have beta not equal to 1. So, T must involve benchmark timing, which we did choose to ignore. For (d), I can see in Figure 1.18 (p. 121 of the Q&A Book) that SR of T > SR of P* (just draw a line from the riskless asset to each of T and P* and look at the slope), so (d) is false.
- Q294/Q266 The reference portfolio on the frontier always has beta=1 (like the beta of M in the CAPM). So, look at the lower plot and find the return on the asset with beta=1. It looks like 12.5% to me.
- Q300/Q272You can do this in your Q3.2.2 (Active Alpha Optimization; p. 254 Q&A Book) spreadsheet to see what happens. I told you in the Q3.2.2 instructions that if you lower risk aversion, the optimizer will trade much more. So, ignoring T-costs, if you lower the risk aversion, you should see that the optimizer chases alpha without regard to risk or T-costs. Ask yourself, what would you do if you faced no T-costs and suddenly your risk aversion dropped? ... You would trade more! Answer (d).
- Q301/Q273 You might not have seen this concept expressed in terms of the cumulative standard normal function N(.). Stock market index returns have fat tails relative to a normal distribution with the same mean and variance. We saw that clearly in Figure 1.16 on p. 71 of FFSI, and we lived through it in 2020:Q1. We also know that broadly diversified major stock market indices have a standard deviation of returns of about 1%. This means that returns beyond 2.5% in either direction are beyond plus and minus 2.5 standard deviations. All this question is asking is about whether the probability mass in the tails of Figure 1.16 beyond plus or minus 2.5% is more or less than you would find beyond plus or minus 2.5 in a standard normal distribution. Given fat tails, the answer is more. The quantity 2[1-N(2.5)] is simply the probability mass in the tails of the standard normal distribution beyond plus or minus 2.5. So, we want answer (a). Any exam questions in 2020 would not use the cumulative standard normal in this way.
- Q302/Q274 asks about |R|<= 1%, that is, small moves in the stock market, of magnitude less than or equal to 1%. I need to rephrase this question slightly more clearly. We know from the peakedness argument that small moves in diversified broad market equity indices of developed countries are more likely than would be seen in a normal distribution with the same mean and variance. We can see that clearly in Figure 1.16 on p. 71 of FFSI. A normal distribution has 68% of its probability mass between -1 an +1 standard deviation. So, our market index must have notably more than 68% mass in this zone, answer (c).
- Q304/Q276 is discussed pp. 318-320 in FFSI.
- Q305/Q277 You are told that the call option is at-the-money. So, given your intuition, the delta must be about 0.5, answer (c), and you are done. No calculation needed. You care if you are a trader, because this tells you roughly how the option changes in value with a change in the level of the underlying. You care if you are an options market maker because the delta tells you how to hedge the option if you just sold it to a customer. Out of curiosity, I just plugged these inputs into the MERTON-II spreadsheet, and I got delta = 0. 4945. So, our guestimate of 0.50 is very close!
- Q308/Q280 You invest $3,000 initial investment. You borrow $7,000 from your broker. You buy $10,000 of stock. The price drops 25%. Oh no. Now your stock is worth only $7,500 and you are scared. You close out the position. You have enough money to pay back the $7,000 loan from your broker. You have only $500 left over. Your return=(final-initial)/initial=(500-3,000)/3,000=-83.33%.
- Q310/Q282 This question is like the mirror image of Q144 (see alsoQ187). Answer (a) is not relevant because the question is about stepping away from the benchmark, but Answer (a) is about the benchmark itself. Answer (b) is correct, but we did not emphasize it in 2020. Answer (c) is correct. Technically, there is a word missing from Answer (c), It should say "Because most *institutional* asset managers do not engage in benchmark timing, and neither will we." If you have a good big picture overview, it is clear from the context, because an active alpha optimization is typically going to be an *institutional* asset management technique, but I will reword that in the next edition. Therefore Answer (e) is correct.
There is an underlying stylized fact here, which is that for the most part, the empirical evidence suggests that benchmark timing (also called market timing) is not profitable. Famous fund manager Peter Lynch described benchmark timing as "futile". His job, as he saw it, was to do deep research on stocks, and then to over-weight the good ones, underweight the bad ones, and seek to outperform the market whichever way it went. So, when the market went down so did his fund, and he did not fight it. One of the main reasons why benchmark timing is futile is breadth. There is a single asset (the benchmark portfolio) and betting on one asset has no breadth. The FLAM (p. 304) says, however, that breadth is very important in determining IR (Share ratio of active returns). So, why chase this one asset when the market is full of so many individual stocks providing us with much breadth?
- Q311/Q283This is under the old KiwiSaver rules. These back-of-the-envelope TVM calculations are all done with approximations. See Section 2.2.3. of FFSI
- Q315/Q287 If you sold an option on one share, then you trade delta shares of stock to hedge it. If you sold an option on N shares, then you trade N*delta shares of stock to hedge it. If delta is positive, then you buy stock to hedge. If delta is negative, then you sell stock to hedge.
- Q320/Q292. This was part of an NZX exploration to find sensible, and small enough, tick sizes (also called the "price step" or the "minimum allowable price variation"). The experiment took place maybe 10 years ago. It led the NZX to where we are now, with reduced tick sizes in some of the more liquid stocks. They should have done it sooner, because it did reduce relative bid-ask spreads. Further reduction in tick sizes, at that time, was likely to have little effect. Increasing algorithmic trading over the last few years may mean, however, that a further reduction in tick sizes could be beneficial in some very liquid stocks. Reducing tick sizes to 1/10th of penny may be too far and could be bad. There are two reasons why a very small tick size could be bad. Traders like to do deals quickly. The finer the price resolution, the more room there is for minor and unimportant price differences in limit order limit prices. Coming to an agreement on price can take longer as a result. Also, you may put in a perfectly good limit order to buy, but then I jump in one-tenth of a penny ahead of you, and this annoys you. You would rather I wait in line behind you at the same price. So, it annoys some traders because it makes jumping ahead of them too easy.
- Q325/Q297 You are given that inflation is 20 bps per month. So, that is the number to use. The annual numbers are APRs with monthly compounding. So, you have R=0.005 per month. g=0.002 per month. PV=2,000,000. N=30*12=360. You want to solve for C, the cash flow, in a growing annuity.
So, PV=(C/(R-g))*[1-((1+g)/(1+R))^N]=C*(1/(R-g))*[1-((1+g)/(1+R))^N]
So, C=PV/{(1/(R-g))*[1-((1+g)/(1+R))^N]}
=2,000,000/{(1/0.003)*(1-((1.002)/(1.005))^360)}
=2,000,000/219.70769=$9,103
- Q326/Q298 If S(t) is very high relative to X, then S(t)/X is big, so ln(S(t)/X) is big, and thus d1 and d2 are big. Recall that the cumulative standard normal function N(.) increases smoothly with its argument. N(-big number)=0, N(0)=0.5, N(big number)=1. So, then c(t) approx= S(t)-e^{-r(T-t)}X=S(t)-PV(X). Basically it means that deep in-the-money call options are priced using a nearly linear equation in S(t). It is like the equation y=bx+a, where y=c, x=S, b=1, and a=-PV(X). This can be illustrated on a plot, but I think I deleted that page from your abridged edition.
- Q333/Q305 This follows from Section 2.1.2 Merton and the Standard Errors of Means and Variances. It is driven by the relationship between variance of returns calculated over different time intervals. It is unstated, but these must be continuously compounded returns, not simple net returns.
- Q342/Q314 This one is less quantitative than it looks. Remember that growth stocks get bid up in price relative to recent earnings because investors think that the stocks have good opportunities for growth in earnings generated by positive NPV projects and retention of earnings. This means that a significant slice of a growth stock's current price is driven by this "PVGO" term (i.e., the present value of future opportunities for growth in earnings, fueled by positive NPV projects and retention). This bidding up of price for growth stocks, relative to recent earnings, gives, by definition, high P/E ratios. So, that means that high P/E ratios go hand in hand with a high proportion of current price P being attributable to this PVGO term. That is, other things (like P and r) being equal, a high-P/E stock will have its price bid up relative to earnings and will have a higher proportion of its price attributable to PVGO, when compared with a low P/E stock. It must be answer (a).
- Q348/Q320 (see also Q288/Q260) This is an opportunity to test your Bachelier knowledge. This is from an actual job interview (it is from several job interviews in fact; it is quite common), but the interviewer did not give any hints. The interviewer said only "You are an options dealer and you just quoted $10 per share as the price for a two-month call option and now the customer is calling back and wants a quote on an otherwise identical three-month option" and they usually say something like "you have 10 seconds to give the quote". Well the only thing that changed is the time to maturity, which increased by a multiplicative factor 1.5. That time to maturity term appears under a square root sign. So, the option price increases by a multiplicative factor of sqrt(1.5). The square-root function is almost linear near 1, so in an interview, I would guess sqrt(1.5) is approx 1.25, but the curvature brings it down a little, and 12*12 =144, so it has to be a little higher than 1.20. So I would guess sqrt(1.5)=1.225, which gives exactly answer (a). Of course, if you have a calculator, you can just calculate sqrt{1.5) exactly, and you do not have to estimate it.
Option trading aside I cannot overemphasize how important this intuition is when trading options. I may be seeking a quick increase in stock price, but I am usually worried almost equally about changing volatility and changing time to maturity (time decay). The Bachelier formula says that an at-the-money call (or put) option is linearly priced in volatility (sigma), and non-linearly priced in time to maturity. This takes us right back to the roots of the valuation in the random walk in Equation 2.2 on p. 149 of FFSI, where the diffusion coefficient has sigma*sqrt(tau), just like in the Bachelier formula.
For example, suppose you see a stock that has collapsed in price because of some short-term bad news. You think the stock will recover quickly. Maybe the uncertainty is high, and sigma just jumped from sigma=0.40 to sigma=0.80. So, you buy a six-month at-the-money call option, betting that the stock will rise soon. Well, what happens if the stock price goes nowhere for a couple of weeks, but the uncertainty dies away and sigma drops back down to sigma=0.40? Bachelier says, correctly, that the option price halves. You lost half your money in two weeks even though time decay had little effect and the stock price did not move. Like I said before, trading stocks is like playing with fire, and trading options is like throwing gasoline on the fire. Do not trade options unless you fully understand them.
I created a spreadsheet about Bachelier option pricing approximations Q257-Q320-20200610.xlsx for the curious.
- Q353/Q325 RS(GPG)=(0.680-0.675)/0.6775=73.8 bps. RS(WBC)=(37.0-36.5)/36.75=136bps. So, one is not 100 times the other. Answer (b) is correct.
- Q357/Q329 hi is a column vector of weights in a portfolio that contains only stock i. The weights have to add to one. So, hi ha s 1 in the ith position and zeroes otherwise.
- Q360/Q332 First draw a time line from 65 to 95. Annual withdrawals. First is at 65, last is at 95. I count N=31 cash flows. R=0.05 (That seems low. Oh, I see, she is at retirement, so she is choosing a relatively safe investment fund). g=0.03 ( a bit more than inflation, most likely). I need to use the Present value growing annuity due (PVGAD). Don't forget the (1+R) multiplier:
- PVGAD=(1+R)*(C/(R-g))*[1-((1+g)/(1+R))^N]=C*(1+R)*(1/(R-g))*[1-((1+g)/(1+R))^N].
- That is, C=PVGAD/{(1+R)*(1/(R-g))*[1-((1+g)/(1+R))^N]}
- =$2,000,000/{1.05*(1/(.05-.03))[1-((1.03)/(1.05))^31]}
- =$2,000,000/23.576889=$84,828.83, answer (a).
- If you got this wrong, I am guessing you did not draw a timeline, did not count N correctly, or failed to include the (1+R) factor. These are the most common student TVM mistakes.
- Q366/Q338 We did not go looking at the few smallest stocks. Often they are so illiquid that they do not even have both a bid and an ask. So, their relative spread is not even able to be calculated.
- Q368/Q340This ETF contains 8410 STOCKS over 50 countries with an expense ratio of only 8 bps per annum:
https://bigcharts.marketwatch.com/quickchart/quickchart.asp?symb=VT&insttype=&freq=&show=
but this ETF contains GOLD and nothing else (it is neither diverse, nor following stocks):
https://bigcharts.marketwatch.com/quickchart/quickchart.asp?symb=IAU&insttype=&freq=&show=
This ETF contains T-BILLS and nothing else (it is neither diverse, nor following stocks):
https://bigcharts.marketwatch.com/quickchart/quickchart.asp?symb=BIL&insttype=&freq=1&show=True
This ETF contains SILVER and nothing else (it is neither diverse, nor following stocks) :
https://bigcharts.marketwatch.com/quickchart/quickchart.asp?symb=SLV&insttype=&freq=1&show=True&time=8
and this ETF has an expense ratio of 968 bps per annum (yes, 9.68% per annum is deducted in fees):
https://www.vaneck.com/etf/income/bizd/overview/?country=US
So, it is not true that all ETFs passively track a stock index, are diversified, and have low fees.
- Q373/Q345 Relative spread (RS) is given by RS=(ask-bid)/m, where m=midspread value. RS=(3.27-3.25)/3.26=0.00613. That's 61.3 bps, answer (b).
- Q376/Q348 Note that Q3.2.2 (Active Alpha Optimization) appears on p. 254 of the Q&A book. Answer (c) looks about right in that case, but there was less spread in the 2020 data used by my Otago University FINC302 students, with the relative spreads varying from 56 bps to 613 bps.
- Q382/Q354: One-sided and two-sided turnover are discussed on p. 300 of FFSI.
- Q384: A full answer to Q384 appears in the box on p.302 of the 13th edition of FFSI
- Q385/Q357 Draw a time line! Count the payments: N=26, R=0.06, g=0.04, PV=$1,500,000. Then use Present value growing annuity due (PVGAD
PVGAD=(1+R)*(C/(R-g))*[1-((1+g)/(1+R))^N]=C*(1+R)*(1/(R-g))*[1-((1+g)/(1+R))^N].
That is, C=PVGAD/{(1+R)*(1/(R-g))*[1-((1+g)/(1+R))^N]}
=$1,500,000/{1.06*(1/(.06-.04))[1-((1.04)/(1.06))^26]}
=$1,500,000/20.700916=$72,460.56, answer (b).
If you got this wrong, I am guessing you did not draw a timeline, did not count N correctly, or failed to include the (1+R) factor. These are the most common student TVM mistakes.
- Q386/Q358 (see also Q419/Q391) On Q3.2.1 (Markowitz; p. 251 Q&A Book) you drew Markowitz frontiers. All through Merton's Markowitz efficient set mathematics in Section 2.6.4 (which you implemented) you will see V^{-1}. This is the inverse of the VCV. So, in order to draw the Markowitz frontiers, you need to be able to estimate the VCV V (using =COVAR(.,.) in Excel), and you need to be able to invert it (using =MINVERSE(.,.) in Excel). Note that invertibility means both that V^{-1} can be calculated and that V*V{-1} is the NxN identity matrix. If you have T (number of time series return observations) fewer than N (number of stocks), however, then even though the VCV is able to be estimated (assuming T is at least 2), V will not be invertible. In the case of 100 stocks and 4 months of returns, you have N=100 and T=about 84. So, V is estimable, but not invertible. So >Q386/Q358 has answer (b) and Q419/Q391 has answer (b).
- Q388/Q360 The Ledoit-Wolf (2004) VCV that we used on Q3.2.2 (Active Alpha Optimization; p. 254 Q&A Book) is the average of two VCVs. These two are the sample VCV and the constant-correlation model VCV (See Equation 2.99 in FFSI). These two VCVs have the same diagonal elements. That is, these two VCVs both just have the regular variances on the diagonal. For example, if the (1,1) element of the sample VCV was 0.02247 (variance of returns to AIA; implying a standard deviation of about 15% per annum if you square-root it), but the (1,1) element of the constant correlation model VCV was also 0.02247 (the same variance of returns to AIA), then when we take an average of two terms from the diagonals of these matrices, the diagonal elements do not change
(because the average of 0.02247 and 0.02247 is still 0.02247). It is
only the off-diagonal elements that differ between these VCVs. That is,
the constant correlation model is a structured model of the off-diagonal
terms. That is, the Ledoit-Wolf VCV and the sample VCV agree on the
diagonal terms, which are just regular variances, and disagree on the
off-diagonals (i.e., all the covariances). You can see this in action in
the Q3.2.2 (Active Alpha Optimization; p. 254 Q&A Book) spreadsheet when you flip between matrices.
- Q390/Q362 This is the definition of relative spread
(RS) RS=(ask-bid)/m, where m is the mid-point of the spread. So,
RS=(6.34-6.25)/6.295=0.09/6.295=1.4297%
- Q418/Q390 Let's stick in some dollar numbers to make it more concrete. Any starting value will give the same answer. Let us use $1000.
Suppose I invest $600 in stocks and $400 in bonds at t=0, total value $1000.
By t=1 (end of Day 1), I have $600*(1 + 0.05) = $630 in stocks, and $400*(1 - 0.025) = $390 in bonds, total value $1020.
By t=2 (end of Day 2), I have $630*(1 + 0.05) = $661.50 in stocks and $390*(1 = 0.00) = $390 in bonds, total value $1051.50.
So, my portfolio value goes $1000 --> $1020 --> $1051.50. The
SNR on Day 2 is R=(Final-Initial)/Initial =
(1051.50-1020.00)/1020=3.08823% approx 3.09%, Answer (d).
Alternatively, you can find weights at each point in time. So, t-1
the weights are h1=630/1020, h2=390/1020, and you can apply these to the
returns R(S)=0.05 and R(B)=0.00 on Day 2 to get: R=h'(R(S)
R(B))'=(630/1020)*0.05+(390/1020)*0.00=3.08823% approx 3.09%, Answer
(d).
Alternatively, it can be done entirely algebraically, and then plug in h1, h2, R(S), R(B) at the end.
- Q419/Q391 (see also Q386/Q358) On Q3.2.1
(Markowitz; p. 251 Q&A Book) you drew Markowitz frontiers. All
through Merton's Markowitz efficient set mathematics in Section 2.6.4
(which you implemented) you will see V^{-1}. This is the inverse of the
VCV. So, in order to draw the Markowitz frontiers, you need to be able
to estimate the VCV V (using =COVAR(.,.) in Excel), and you need to be
able to invert it (using =MINVERSE(.,.) in Excel). Note that
invertibility means both that V^{-1} can be calculated and that V*V{-1}
is the NxN identity matrix. If you have T (number of time series return
observations) fewer than N (number of stocks), however, then even though
the VCV is able to be estimated (assuming T is at least 2), V will not
be invertible. In the case of 100 stocks and 4 months of returns, you
have N=100 and T=about 84. So, V is estimable, but not invertible. So Q386/Q358 has answer (b) and Q491/Q391 has answer (b).
- Q421/Q393 Under these traditional US margining
requirements ,if you have $1 then you can go at most long $1 and short
$1. So, that would give answer (a). NZ rules are more lax. In NZ you can
walk into this with only $0.50 and go long $1 and short $1. We did this
when I was a practitioner, and I have done it in my own brokerage
account (as described on p. 230 of FFSI), and I can explain the NZ-US
margin rule differences with some simple examples.
- Q425/Q397 Just rearrange [position
margin]/[position value]=0.002 to solve for position value, using
position margin = $1000. This degree of leverage is so speculative that I
do not classify it as investing anymore.
- Q431/Q403 The problem is that the only way you
could find the weights for the frontier portfolio was to go to the end
of the time period and look back (like in Q3.2.2; Active Alpha Optimization). So, you could not have recommended it in advance.
- Q444/Q416 Note that one-twenty-fifth is 1/25 which is 0.04. So, I would find one basis point of $1,000,000 and then divide that by 25.
- Q453/Q425 (see also Q133) SNAP: A
student asked "why does T-Costs = 10 + (bid/ask spread)/2?" If you paid
$26.05 and paid $10 commission where does the extra T-Cost come into
play in reality? My answer is ($10 commission +100*half-spread)=$10+100*0.025=$12.50 T-costs. Purchase price = 100*$26.05=$2,605. So ratio is $12.50/$2,605= about 48 bps.
As a former practitioner I can tell you that the width of the bid-ask
spread is really important. More so than commissions when you trade in
large quantity (as most practitioners do). The wider the spread, the
more costly it is to trade! Remember those average NZX spreads? The
width of the spread changes when you might want to trade during the day.
That's why it is the third component in the objective function you
optimize on the active alpha optimization function (Equation 2.81 on p.
290). Similarly, see these FX spreads measured in pips: https://www1.oanda.com/forex-trading/markets/recent Pick
some currency pair you are more familiar with if you want, like NZD/USD
and see how variable they are. There are some times when you definitely
do not want to trade. If you are a retail investor buying 100 shares,
you likely don't care, but spreads are a very important T-cost to
practitioners.
You have to pay this half-spread. It is unavoidable, but it is part of the $2605. It is not extra. You have to think like a trader. So, let me rephrase the question. Suppose you want to buy 100 shares. You know the commission is going to be $10.
On Monday morning, the spread is 26.00-26.05 (mid-spread price = 26.025)
On late Monday afternoon, the spread is 25.90-26.15 (mid-spread price = 26.025), perhaps because there is less liquidity, but no change in asset fair value.
At which time, morning or late afternoon would you rather have placed your trade? In both cases, the fair value of the shares was 26.025. In both cases the commission was $10.
In the morning, you end up paying fair value of $2,602.50 + the half-spread of $2.50 = a total of $2,605.00.
In late afternoon you end up paying fair value of $2,602.50 + the half-spread of $12.50 = a total of $2,615.00.
Notice that the commission did not change, and the mid-spread value
did not change. If you said you want to trade in the morning, it is
because there is more liquidity, a narrower spread, and therefore lower T-costs.
The difference in what you pay between the two scenarios is not because
the commission changed or because the fair value changed. It is because
liquidity changed, and widened the spread, and that is a T-cost. All I
am doing is quantifying that part of the T-cost, because traders care
about it and it affects when and how they trade.
- Q454/Q426 (see also Q268/Q240)
This is a growing annuity problem. You have to compare the future value
of two growing annuities (let me call them FVGA9 and FVGA8 for the two
rates R=9% and R=8%). Before doing any calculation, my gut instinct is
that it must be the number close to 35%. From Chapter 1 of FFSI, for a lump sum investor, an extra 1% increases final wealth for you folks (say 45 years to retirement) by 50%, and for an annuity investor, an extra 1% increases final wealth for you folks by about 30%.
Both have the same growth rate g=4%. 1. Draw a timeline. Actually, you need two timelines, one for 9% and one for 8%! 2. Write down algebra for FVGA9/FVGA8. 3. Cancel out the first cash flow (which is the same in each and cancels in this ratio). 4. Stick in numbers and calculate the ratio.
Here are the details: FVGA(C,R,g,N)=(C/(R-g))[(1+R)^N-(1+g)^N]. So, I
need to take the ratio FVGA(C,9%,4%,50) over FVGA(C,8%,4%,50). Drop
the C because it cancels. The numerator is
(1/(.09-.04))*[1.09^50-1.04^50] and the denominator is
(1/(.08-.04))*[1.08^50-1.04^50]. I get 1345.0167/994.8732=1.352, a 35.2%
increase.
- Q463/Q435 Marketable limit orders were discussed in Section 2.4.1. A marketable limit order to buy is a limit order to buy that can be immediately executed.
So, there must be sufficient depth on the ask side of the CLOB (though
not necessarily at the best ask; it could be deeper). (a) is not correct
because it says it waits; (b) is not correct because it says it waits
(wrong) on the ask side (wrong). (c) is wrong because it says it waits
(wrong) on the bid side. (d) is wrong because it says that it waits
(wrong) on the bid side. (e) is correct. None of the above.
- Q465/Q437 This was an exam question given on Tsunetomo's birthday! Now it is Q465/Q437 in the Q&A book. Did you draw a timeline? You have to draw a timeline! This is the PV of an ordinary annuity due (PVAD). N=26 (common error is to mis-count). Don't forget (1+R) because it is an annuity due (another common error). PVAD=(1+r)*(C/R)*(1-(1+R)^-N)=1.05*($200,000/0.05)*(1-1.05^-26)=$3,018,788.9
- Q471/2020MTQ29 (see also Q473 for
extra practice) Is a retirement savings exercise. It is realistic in
that you save a fixed proportion of salary on a regular basis. It is
unrealistic in that it is annual; most people save weekly,
fortnightly, or monthly. You must draw a timeline for any TVM problem. I
have graded thousands of long-answer TVM exam questions and students
with timelines always do best. I cannot draw a timeline here easily.
- Q473 See Q471/2020MTQ29.
- Q474/2020MTQ30 You invest $400 of your money.
You borrow $600. You buy $1,000 worth of stock. The stock rises by 35%
from $10.00 a share to $13.50 per share. You unwind the position. What
was your return? There are different ways to work this out.
- FIRST We argued several times in several places (e.g., the margin leverage box on p. 215 of FFSI, the Chinese Farmer Video*)
that you just need to find the "multiplicative factor" and then we just
multiply the underlying return (35% here) by this factor.
- This multiplicative factor is 1/[margin rate] where [margin rate]=[position margin]/[position value]=[how much of your money you invested]/[total cost of position].
- In this case, the margin rate = $400/$1000 = 0.40.
- So, the multiplicative factor = 1/0.40 = 2.50.
- So, if the stock went up by 35%, then your leveraged position went up by 2.50 times this. That is, your rate of return is R = 2.50 * 35% = 87.50%, answer (g).
- SECOND We can instead work it out form first principals. R=(final-initial)/initial, where initial is the initial investment (i.e., what you took out of your pocket), and final is the final is the value you get back.
- In this case initial = $400. So, we need to find the final value.
- Well, we bought $1000 worth of stock, and it went up in value by
35%. So, we end up with $1350. That's not our final value though,
because we borrowed $600 that we need to pay back. After paying that
back, we are left with $1350-$600 = $750. So, final = $750.
- Thus R=(final-initial)/initial = ($750-$400)/$400 = 87.5%, answer (g).
*Chinese Farmer Video*: Go to the three-minute story (NBR July 29, 2015) at 19:18 mark on this video.
He has USD165,000 (it's a US news show, so they convert everything to
USD). He also invests some money from family, bringing his total to
something less than USD200,000. Then his broker...
- Q475/2020MTQ15 Draw a timeline. I will
withdraw $75,000 at t=1. I will adjust it for inflation to withdraw
$75,000*(1+i) at t=2, and $75,000*(1+i)^2 at t=3. Using i=0.015, I get
$75,000*(1+i)^2=$75,000(1.015)^2=$77,267 to the nearest dollar. This is
Answer (e).
- Q476/2020MTQ14 The Bengen approach (pp. 333-337 of
FFSI) is based on many simulations of possible investment return
scenarios during your retirement. Bengen looks for a max
proportion you can withdraw from your initial wealth (assuming that you
then adjust that dollar amount each year for inflation, to keep it
constant in real terms). This proportion must keep you safe
from financial ruin most of the time (which usually means in about 95%
of simulated futures). He finds that if you are 60-65, a safemax
4% number is about right. It is a very well known and widely used
retirement wealth withdrawal rule. Our TVM approach, however, used only
mean returns. So, the TVM approach tells you what wealth you need to
meet projected expenses if you will experience mean returns every year,
but the Bengen approach says no, we almost never get an average year in
the markets. So, we need a larger amount of wealth that protects us from
financial ruin over most possibly simulated futures, which includes a
spread of possible returns during your retirement. We want to be safe
from ruin in all but the 5% worst cases, not just for an average case.
So, that takes a much bigger number. This is Answer (b).
- Q481/2020MTQ41 is about problems with the traditional VCV. Discussed in detail in Section 2.8.1 on pp. 311-312.
- Q482/2020MTQ42 In Q3.2.1
(Markowitz) I gave you a vector "mu" of mean returns on stocks, and a
variance-covariance matrix "V" and you used Merton's mathematics
(Equations 2.50-2.55) to draw the Markowitz frontier, the Tobin
frontier, and the tangency portfolio. You also plotted the benchmark
portfolio B inside the frontier. I argue in a
Black-Litterman intuition document
(prepared for my students in 2020) that Fischer Black had no faith at
all in our estimated mean returns, because of mean blur (a constant
theme in this course). He did, however, have faith in the CAPM. He
wanted to draw the same picture, but he did not want to use our mu
vector calculation. Instead, we worked backwards and deduced what the vector of mean returns would have to be to make your Q3.2.1
(Markowitz; p. 251 Q&A Book) picture look like the Markowitz-CAPM
world (i.e., where B and T are the same, and behave like "M" in the
CAPM, and sit on the Markowitz frontier at the tangency point). I did
the math too, and I figured that the vector mu must be given by my
Equation 2.82. Black called these "equilibrium implied mean return
estimates" because they are consistent with a CAPM-type equilibrium.
In Section 2.7.13 of FFSI we took this analysis one step further and
we said OK, let's use the Black-Litterman equilibrium estimates of mean
returns, but add our Grinold-Kahn alphas to them to get skilled
forecasts of returns. Then we used those skilled forecasts to locate a
new tangency portfolio and we took that as our optimum GK-BL portfolio. I
found in a 2020 exercise with students that this portfolio outperformed
our benchmark portfolio by 20 percentage points over the following
year. I tested this approach with one other previous year of data, and
it outperformed similarly. Wow!
- Q487/2020FINQ22 You must draw a timeline. Beware of
the "due" part of the annuity.
PV of growing annuity due (PVGAD). PV=$2,500,000, N=21, solve for C,
R=0.04, g=0.02.
PV=PVGAD=(1+R)*PVGA=(1+R)[C/(R-g)][1-{(1+g)/(1+R)}^N]. So,
C=PV/{(1+R)[1/(R-g)][1-{(1+g)/(1+R)}^N]}=2,500,000/[17.413483]=$143,566.91.
Then deflate C/(1+i)^43=$61,269.87 for i=0.02=inflation. Gives answer
(e).
- Q488/2020MTQ37 Regarding the SML, there are only a few things to remember:
1. The SML is a plot of betas on the x axis versus returns on the y axis.
2. Betas are always calculated relative to some index. For example, beta of stock i relative to the benchmark index B.
3. Beta in the CAPM is a relative covariance:
beta(i)=cov(R(i),R(B))/var(R(B)), say, for beta of stock i relative to
index B. We executed variances and covariances using matrix notation, as
appearing in Q37 and as introduced on p. 255 of FFSI, for example, cov(R(i),R(B))=hi'VhB, and var(R(B))=hB'VhB.
4. If your index is on the Markowitz frontier, the SML is a perfect straight line. If not, the SML is a jumble of points.
That's it. That's all you need to understand.
- In the theoretical CAPM, "M" is on the frontier, so the SML is a straight line.
- In Q3.2.1 (Markowitz; p. 251 Q&A Book) you calculated betas relative to B (B was not on the frontier and the SML was a jumble).
- You also calculated betas relative to T (T was on the frontier and the SML was a perfect straight line).
- In Q37, the index (I called it C) was on the frontier. So, the SML will be a perfect straight line.
Note that Roll (1977) argued that you cannot test the CAPM by doing what we did on Q3.2.1
(Markowitz; p. 251 Q&A Book) (i.e., collecting average returns and
betas and seeing if they are related linearly). That's because whether
you get a linear relationship between average returns and betas depends
100% on whether the index portfolio is on the frontier or not, and 0% on
whether the CAPM holds in the world.
- Q489/2020MTQ43 Says it uses Black-Litterman
returns. By definition, the Black-Litterman returns put the benchmark
portfolio on the frontier. They are designed for that purpose. Then,
because B is on the frontier, you must get a perfect straight line for
the SML. Just go back to Q3.2.1 (Markowitz; p. 251
Q&A Book) and copy and paste the Black-Litterman returns over the
top of the vector of mean returns mu I calculated, and you will see that
the SML is linear for betas relative to B.
- Q506/2020MTQ19 You sold an option to a customer.
You are short the option. You are said to have "written" the option. To
hedge your exposure, you need to synthesize an offsetting hedge
position. In this case, the hedge is a long position in an option. So,
you need to synthesize a long position in an at-the-money call option.
You need to buy Delta shares of stock for each share covered by the
option. Delta is about 0.5 for an at-the-money call option. So, you need
to buy about 50 shares of stock to hedge.
Further details after follow-up questions from students:
Please read the following extract from Otago University FINC302 Problem Set 3 from 2020:
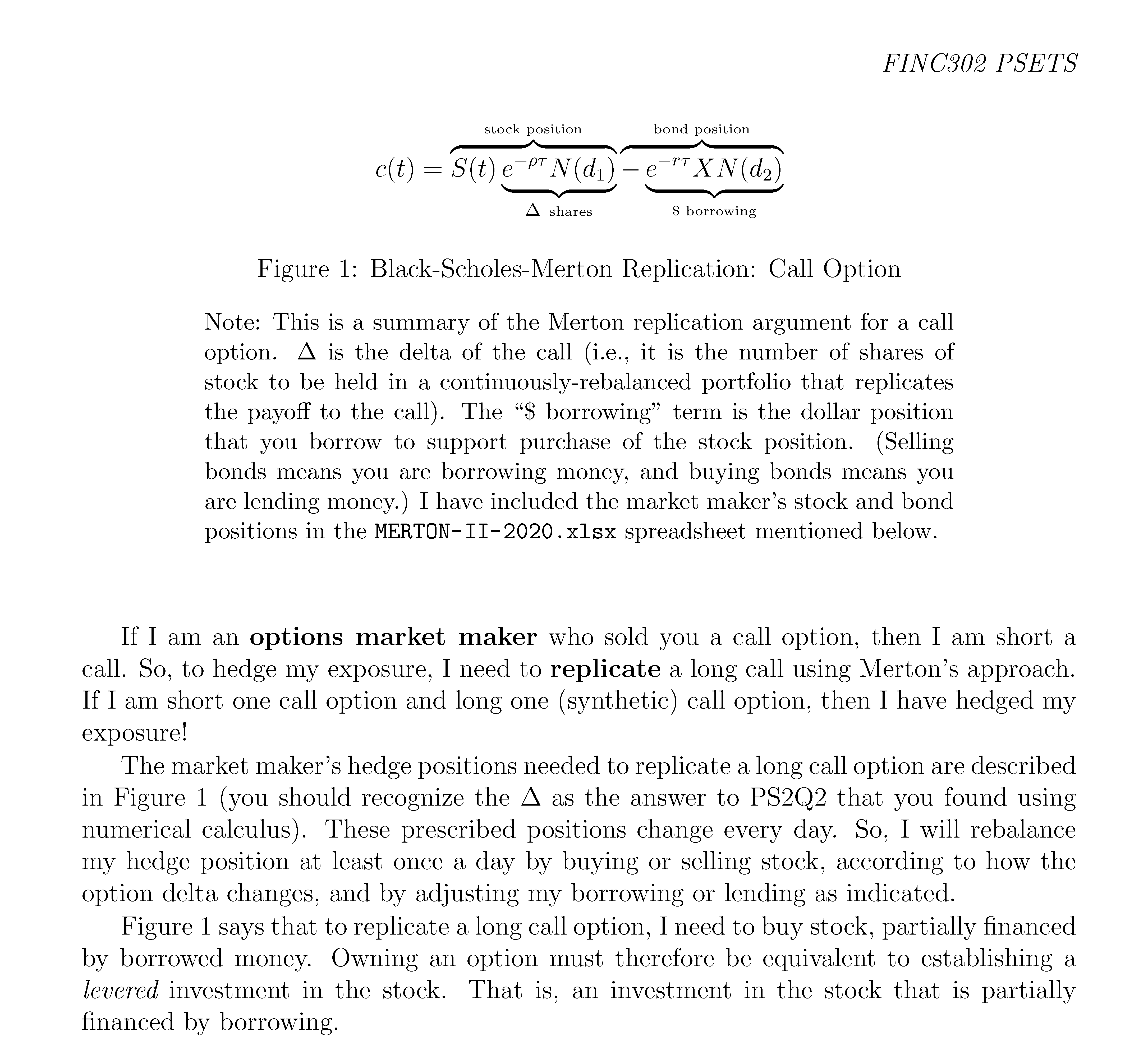
This is all expressed in terms of an option on one share of stock.
So, if you sell an option on one share of stock, then you are short
an option on one share of stock, and you hedge that exposure by
replicating a long option exposure using this formula. So, you must buy delta shares of stock.
If the option you sold covers N shares of stock, then you hedge by
buying N*delta shares of stock. So, if N=100, and delta=0.5, then you
buy 50 shares of stock.
If the option we were dealing with was out of the money, but not
terribly far out of the money, then the delta would be about 0.3 or 0.4,
or thereabouts in that case. If the option were in the money, but not
terribly deep in the money, the delta might be 0.6 or 0.7. Indeed, you
can see in the first plot in the bbsGREEKS spreadsheet that the slope
(i.e., delta) varies from 0 for a deep out-of-the-money call, to about
0.5 for an at-the-money call, to about 1 for a deep in-the-money call.
I have several practice questions in the Q&A Book, but most
involve put options, which I said I would not trouble you with in an
exam. Sometimes I just give the delta, sometimes I ask you to estimate
it given the moneyness of the option, and sometimes I ask you to
calculate it as a numerical derivative.
- Q511 You are told that you rebalanced just once, moving from portfolio B to portfolio P. So, the
quantity |hB-hP|'i is just two-sided turnover as a proportion of portfolio value, asnwer (c). The quantity
"total active position" mentioned in (e) is not defined. I typed it into Google using quotes, and got only two hits,
neither of which was relevant. There is, however, a quantity called "active share," which is 0.5*|hB-hP|'i,
as
discussed in the last paragraph of Section 2.14.8 of FFSI (pp. 366-367
of the 11th edition). If answer (e) had said "it is twice the active
share,"
then (e) would also have been correct.
- Q519/Q444 The math uses the cumulative standard
normal function. I don't think you can even do this calculation in
Excel, because Excel cannot calculate probabilities this small. The
short answer to your question is that if the stock returns were normally
distributed with the same mean (about 3 bps) and same standard
deviation (about 100 bps = 1%) as the empirical data, then a move of 10
standard deviations will never happen in your lifetime, or even in one
billion of your lifetimes. It's basically impossible. Read pp. 68-73 of
FFSI, and look at Table 1.7 on p. 69 of FFSI.
An informal way to think about this is to look at Figure 1.16 on p.
71. That normal distribution curve goes to zero so quickly that it
really excludes the possibility of very extreme events. For
moves of 5%, say, then yes, a model using normally distributed returns
says that can happen, though not very often, but once you get past moves
of about 6%, it's just not going to happen in a mathematical model
where stock returns are normally distributed (even though the S&P500
moved this much and more many times udring 2020:Q1).
- Q522/Q447 and Q523/Q448 One way to get the benchmark to be on the frontier, is for two things to happen: If we can use forward-looking returns instead of backward-looking returns and investors are mean-variance optimizers
within this group of stocks. (Technically, we also need that there are
no T-costs and some other perfect market assumptions.) In Q522/Q447, only one of these was mentioned. In Q523/Q448, both were mentioned. If both hold, then we are close to the CAPM assumptions, and we can get B on the frontier, but we need both. That's why Q522/Q447 has answer (e) but Q523/Q448 has answer (e).
- Q524/Q449 We did not emphasize this. If the beta of
asset C is negative, that means the covariance of returns on asset C
with the returns on the market is negative, and that makes Asset C a
diversifier. Investor like returns and dislike risk. They are willing to
pay for assets like this, that diversify (i.e., that reduce portfolio
risky when dropped into a portfolio). In the CAPM world, they are
willing to pay so much for asset C that its current price gets pushed
up, and thus its expected return (given some future expected payoff)
becomes lower even than the expected return on T-bills.
- Q525/Q450 Please ignore this question. It is not
worded properly. I need to edit this and add a sentence that says
something like "Given the Roll critique, what can you conclude about our
efforts to test the CAPM?"
- Q531/Q456Let give a long answer to explain why it is answer (e), none of the above:
After the CAPM was invented in the early 1960s, researchers decided
to test it. Richard Roll published a research paper in 1977 that
criticizes many tests of the CAPM. There are two main parts to his
criticism.
Roll Critique Part 1 The CAPM is expressed in terms
of "M", the world market portfolio of all risky assets. (I tried to
describe it in Figure 3.12 on p. 437, but I left some things out, like
the value of your human capital). You cannot see M. It is not reported
in the financial news or on Bloomberg. If you cannot see M, then you
cannot test the CAPM.
Roll Critique Part 2 Suppose you try to test the
CAPM anyway. The CAPM says that there is a linear relationship between
returns and betas. Suppose you collect mean returns and suppose you calculate sample betas. Suppose you test to see if your returns and your betas are linearly related. This sounds like a test of the CAPM, but is it a test of the CAPM?
Richard Roll says no, this is not a test of the CAPM. On Q3.2.1
(Markowitz; p. 251 Q&A Book) we collected mean returns and we
calculated sample betas and we plotted them against each other to see if
they were linearly related. Roll says, however, that whether returns
and betas are linearly related is driven by only one thing:
- If you choose a reference portfolio on the Markowitz frontier, like T, then your mean returns and your sample betas will be perfectly linearly related.
- If you choose a reference portfolio not on the Markowitz frontier, like B, then your mean returns and your sample betas will not be perfectly linearly related, and you get a jumble of points.
This is a purely mathematical result driven by the mathematics appearing on p. 267 of FFSI. This mathematical result is true if the CAPM holds in the real world. This mathematical result is also true if the CAPM does not hold in the real world.
So, what this means is that whether you find a linear relationship
between mean returns and sample betas is dependent only upon whether
your reference portfolio is on the frontier or not; Nothing else. In
particular, linearity or not of the return-beta relationship is not driven in any way by whether the CAPM holds or not. So, you cannot test the CAPM by collecting mean returns and sample betas and looking to see if they are linearly related.
We saw exactly this in Q3.2.1 (Markowitz, p. 251
Q&A Book). When you calculated betas relative to T you got a perfect
straight line relationship between mean returns and betas. When you
calculated betas relative to B, you got a jumble of points when you
plotted mean returns and betas. This was not a test of the CAPM.
- Q559/Q481 Just find the FV of each investment using the net returns.
- Q561/Q483 Follows from the 17 Facts about P/Es discussion.
- Q562/Q484 Answer (a) is false. It is mutual funds
that you buy or sell directly from the fund company at end of day
prices. Note that the question says "most ETFs". There are exceptions.
For some odd reason, the NZX allows you to buy ETFs directly from them.
This is the only exception to this that I am aware of globally.
- Q563/Q485 Still true in 2021.
- Q571/Q493 Although the dollar numbers have changed,
the proportions have not. Roughly 98.5% of money in ETFs is in passive
ETFs, and roughly 20% of the money in mutual funds is in passive mutual
funds. It must be answer (a).
- Q574/Q496 These Fama-French extra factors are
significant in explaining returns because the market proxy has been
poorly measured. We argued that this (extra factors explain returns) is
exactly what you would expect to find if the original single-factor CAPM
holds.
- Q576/Q498 Funny but true. They "cut the flowers and water the weeds."
- Q579/Q501 One-sided turnover is
the count (or dollar value) of all shares bought. It is also the count
(or dollar value) of all shares bought. These two numbers must be the
same. Two-sided turnover is the sum of these; it counts
buys and sells. In a trading strategy, we usually express turnover as a
percentage figure. That is, the value of the trading as a percentage of
the value of the portfolio. At the end of the extended answer to Q286/Q258 I give a simple numerical example of this for a trading strategy. Now to Q579/Q501. The turnover reported for shares trading on an exchange is always one-sided
turnover. The exchange counts the number and dollar value of shares
that were bought (or, equivalently, they count the number or dollar
value of shares that were sold). They never count both. In this
question, you have the count (1,610,697) and the dollar value ($13,164,799.64), but you also have the reported open, high, low, and close stock prices (around $8.15-$8.20).
If you multiply the count by the stock prices, you get, roughly, the
dollar volume. So, the count must be one-sided. If the count were
two-sided, then the big dollar number would have to be twice as big,
more like $26,300,000.
- Q581/Q503 It's definitional. Each of (b), (c), and
(d) say something false: you cannot earn interest on a borrowed stock,
you do not get dividends, and there is not margin loan here (you
borrowed stock, not cash).
- Q582/Q504 Just use the formula for correlation. corr(3R,R)=cov(3R,R)/[std(R)*std(3R)]=1.
- Q586/Q508 The trend has slowed a little, but it is still happening in 2021.
- Q589/Q511 The quotes are the best bid and the best ask. They are quoted to you by the market maker.